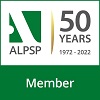
Topical Collection on "Remote Sensing Data Mining for Environmental Change Monitoring using Neural Networks"
Deadline for manuscript submissions: 10 October 2024
Collection Editors
Federal University of Technology, Minna, Nigeria
Email: ajao.wale@futminna.edu.ng,lukmanadewaleajao@gmail.com
Research Fields: Computer Engineering, Computer Vision, FPGA, IoT Security, Blockchain
Chonnam National University, Gwangju 61186, Korea
Email: aliyu@jnu.ac.kr
Research Fields: Blockchain, Computer Network Security, Distributed Computing, Autonomous Networks
Council for Scientific and Industrial Research (CSIR); Advanced Internet of Things (AIoT), South Africa
Email: aonumanyi@csir.co.za
Research Fields: Cognitive Radio, Wireless Communication, Image Processing
Topical Collection Information
Remote sensing has become a crucial tool for monitoring the sustainable environment of data mining areas. Quarrying domains are hotspots for the prospecting and extraction of precious metals and, consequently, for the degradation of the natural world, marked by conflicts between commercial growth and environmental safeguarding. The advancement of remote sensing offers ideal circumstances for monitoring ecological modifications within data mining spots and extensively, quickly, and continually detecting environmental elements. Drought is really regarded as one of the most destructive natural calamities. The harsh effects of drought have an impact on both humanity and the natural world on several levels. Accurate drought prediction and identification of its numerous elements are prerequisites for effective and economic management. Consequently, there is a sizable corpus of knowledge on dehydration monitoring. On the other hand, there has been a noticeable rise in the quantity of data connected to drought, along with a notable expansion of remote sensing databases.
New avenues for environmental monitoring and evaluation have recently become available with the use of artificial intelligence (AI) technologies and data integration methodologies for the analysis of satellite pictures and remote sensing inputs. This is mostly because of the development of data mining techniques, which make it easier to extract valuable information on a wide scale from incompatible and geo-referenced resources. The world's growing inhabitants and human activity are causing a major shift in what we are seeing. Furthermore, the classification issue is typically considered to involve classifying a single picture, although it has not yet been investigated if a single neural network is capable of automatically classifying and extracting features from a set of stored visuals. Using earlier preset weighting factors, neural networks can easily categorise data based on general pattern matches as an alternative to doing individual assessments using analytic logic used for individual data quantities. Consequently, there are many less analytical duties. Nowadays, assessing the environmental effects of mining activities is essential. The recognition, delineation, and monitoring of contaminants and impacted areas, including abandoned land, as well as changes in subsurface utilisation and reservoirs, are made possible by the use of remote sensing data. Several seasons have seen a significant increase in the variety of explores that are striving hard to improve the use of remote sensing for changing observation of the environment and botanical expansion characteristics in the data mining sites.
This Topical Collection aims to compile the most recent and cutting-edge concepts for using neural network algorithms in future environmental change applications. We also encourage presentations that address problems with theoretical models, novel findings in neural network immigration in high-performance computing systems, or intelligent onboard computation.
More specifically, we particularly encourage contributions in the following areas:
- Utilising neural networks, tracking the environment of data mining areas
- Advancements in remote sensing surveillance of the natural surroundings in data mining
- Remote sensing with neural networks fordetection of environmental change
- Enabling environmental compliance evaluation using a neural network with remote sensing
- An evaluation of data mining and remote sensing techniques environmental monitoring
- Data mining integrating monitoring from remote sensing and sustainable environment changes
- Assessing data mining efforts for environmental monitoring through remote sensing
- Using hyperspectral remote sensing in data mining for tracking environmental conditions
- Algorithms for neural networks: a synthesis for remote sensing activities
- Advances in remote sensing for environment, and epidemiological using neural networks
- A cohesive technique for monitoring environmental change impacts and remote sensing