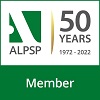
Topical Collection on "Deep Learning for Environmental Monitoring using Satellite Imagery"
Deadline for manuscript submissions: 30 November 2024
Collection Editors
Universitas Widyagama Malang, Indonesia.
Email: aviv@widyagama.ac.id, avivyuniarrahman.id@gmail.com
Universitas Buana Perjuangan Karawang, Indonesia.
Email: aprilia@ubpkarawang.ac.id
Universitas Buana Perjuangan Karawang, Indonesia.
Email: bayu.priyatna@ubpkarawang.ac.id
ORC-ID: https://orcid.org/0000-0002-6207-5185
Topical Collection Information
Deep learning (DL), a popular and cutting-edge computational intelligence framework derived from the conventional neural network (NN), has significantly surpassed conventional models in terms of performance. The intent of the recent developments in deep learning can be combined with accessible multidimensional satellite imagery to identify barriers and gold mines, among other objects, in improved imagery that spans large geospatial areas. There has been an important advancement in creating a DL technique for several environmental science applications. An analysis will be done on the possible applications of DL in environmental remote sensing, which includes knowledge recovery and anticipating, data fusion and reduction, environmental parameter access, and land cover modelling. After that, a standard system for networks will be shown. Next are the DL environmental monitoring programmes.
The most recent free earth-observing imagery is available to everyone, including digital elevation visualisations, meteorological data, and imagery from acoustic and optical satellites. Obtaining an adequate number of satellite images and addressing various related technical challenges, such as cloud cover, choosing suitable spectra stripes, and performing image authorization, are essential for use in satellite imagery. It appears that this is one of the significant drawbacks related to pinpointing outfalls and delineating the limits of windthrow regions. If shadows and the issues they cause can be successfully reduced, then, when choosing photos for satellite imaging, consideration should be given to the angle at which sunlight is incident. In addition to providing open-source image datasets, the deep-learning algorithms are integrated into a pipeline that processes multispectral images and space imagery over huge geographic regions. Deep learning algorithms have gained popularity in environmental science and can be useful in a variety of ecological situations, including resource administration and preservation of the environment, addressing several different ecological challenges. The deep learning technique is relatively new and has great potential in the fields of forestry and vegetation science. There are very few studies identifying unique features of problem resolution with deep learning techniques in the context of the issue of identifying abnormalities in forests. The models are also pre-trained and prepared for use on fresh imagery.
Highlights of this special issue include the access to excellent-quality imagery from satellites and the quick development of computer vision, which have made environmental surveillance and oversight more precise, effective, and scalable. The scientific progression from pixel-classification is based on model-based prediction to deep learning using manual training on satellite images.
Research papers that consider the following questions and themes are welcomed:
- Using imagery from satellites and deep learning to improve environmental monitoring
- Advantages and disadvantages of deep learning in environmental satellite imagery
- Integrating saellite imagery and deep learning for scenario and environment monitoring
- Implementing deep learning to identify tailings dams and extraction in satellite images
- Environmental trend monitoring with deep learning-based identification of changes
- Automated windthrow detection with deep learning and ultra-high-resolution satellite imagery
- Employing deep learning to create cloud-free satellite images from image sequences
- An automated assessment of satellite data using deep learning for environmental management
- Applying deep learning techniques to extremely superior resolution satellite images
- A deep learning method for satellite imagery-based recognition of ships
- Enhancing categorization with deep learning with the Eurosat dataset