Topical Collection on "Advancement in Data Science for Remote Sensing Based Geosciences and Radiosciences for Earth Vision"
Deadline for manuscript submissions: 13 January 2025
Collection Editors
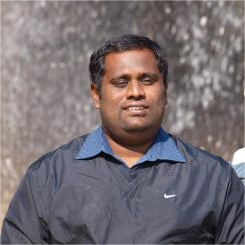
Karpagam Academy of Higher Education, Coimbatore, India
E-Mail: alfreddaniel.j@ieee.org
Interests: VANET; Cooperative Spectrum Sensing; Machine Learning; IoT; AI
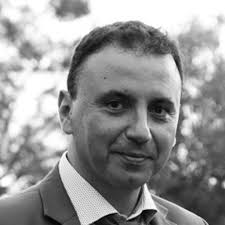
Department of Mechanical Engineering, University of West Attica
E-Mail: sarris@uniwa.gr
Interests: Fluid Mechanics and Magnetohydrodynamics; Turbomachinery; Bioengineering
Topical Collection Information
Dear Colleagues,
Earth observation is a rapidly expanding field of research that brings together Machine learning, computer vision, and signal/image processing. The overall goal is to use data obtained by airborne and spaceborne sensors to give homogeneous knowledge about processes occurring on the earth's surface. Multiple inference tasks, spanning from detection through data mining, multi-resolution, multi-temporal, multi-sensor, multi-modality fusion, and regression, to mention a few, are required for Earth Observation. Location-based services, large-scale surveillance, online mapping services, 3D urban modelling, natural hazard forecasting and response, navigation systems, virtual habitat modelling, climate change monitoring, and other applications are included. Due to the large volume of data, highly automated scene interpretation methods are required.
Remote sensing relies on understanding by capturing electromagnetic radiation from the earth's surface or capturing and analysing other objects in space in the case of Astro informatics. IR measurements, synthetic-aperture Radar, multispectral, optical, satellite imaging, and light detection and range are all common remote sensing data sources. These data are used in various applications, from photography to multi-beam sonars and seismic tomography. In satellite-based geodesy, SAR interferometry and radar altimetry are often utilized. Essential attributes such as spatial resolution, sampling rate, sensor footprint, dwell rate, signal-to-noise ratio, and spectral resolution are used to determine the standard of remote sensing data. Data science is gaining traction in a range of geoscience applications. There is a great desire to learn more about current developments and the promise of data science for geoscience in academia, industry, and government. Land cover classification, precipitation, and soil moisture estimation, yield prediction and crop type detection, spatial downscaling of satellite observations, tropical cyclone intensity estimation, cloud process representations in climate models, and ocean turbulence modelling are just a few examples of ML applications.
Data collected from remote sensing and other sensors, combined with spatially explicit machine learning algorithms, will result in smarter regions and cities, cleaner oceans, productive yet sustainable agriculture, more efficient response to natural disasters, and the reduction of human-caused environmental impact. The efficiency of machine learning approaches that abridges remote sensing with applied and manageable geoscience information is increasingly important in retrieving knowledge through proper data preparation, modelling, validation, and dissemination. Current sensors' high resolution is critical for detecting and modelling fine-grain interactions of spatiotemporal phenomena that characterize climate, land, and environment. More scientific discoveries in geoscience have resulted from the growth of data science, upgrading computational equipment and capacities, and the blossoming of open data and big data in geoscience.
Collection Editors
Dr. J. Alfred Daniel
Dr. Ioannis Sarris
Keywords:
- Remote Sensing
- Earth Observation
- Geoscience Data Science
- Spatial Analysis
- Machine Learning