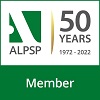
Applications of Artificial Intelligence in Precision Irrigation
DOI:
https://doi.org/10.30564/jees.v6i2.6679Abstract
This paper provides an overview of the various applications of Artificial Intelligence (AI) in precision irrigation. It covers key research areas, methodologies, challenges, and future prospects in the field. The methodology is based on exploring how AI technologies are being used to optimize water management in agriculture and examines the growing body of research on the application of AI in irrigation systems. Deep investigation was conducted to explore how AI technologies can enhance water management in agriculture, leading to improved water management and crop yield in addition to resource efficiency. The paper discusses AI-based methods for monitoring soil conditions, weather forecasting, and real-time decision-making in irrigation. However, integration of AI systems with existing irrigation infrastructure and farming practices can be challenging, requiring significant investment in hardware and software.
Keywords:
Artificial Intelligence (AI); Irrigation; Agriculture; DataReferences
[1] Branca, G., Chileshe, P. Climate change mitigation in the East and Southern Africa region: An economic case for the agriculture, forestry and land use sector. IFAD Research Series 92. Rome: IFAD.
[2] Collins, C., Dennehy, D., Conboy, K., et al., 2021. Artificial intelligence in information systems research: A systematic literature review and research agenda. International Journal of Information Management. 60, 102383. DOI: https://doi.org/10.1016/j.ijinfomgt.2021.102383
[3] Talaviya, T., Shah, D., Patel, N., et al., 2020. Implementation of artificial intelligence in agriculture for optimisation of irrigation and application of pesticides and herbicides. Artificial Intelligence in Agriculture. 4, 58–73. DOI: https://doi.org/10.1016/j.aiia.2020.04.002
[4] Pérez-Blanco, C.D., Hrast-Essenfelder, A., Perry, C., 2020. Irrigation technology and water conservation: A review of the theory and evidence. Review of Environmental Economics and Policy. 14(2), 216–239. DOI: https://doi.org/10.1093/reep/reaa004
[5] Bijekar, S., Padariya, H.D., Yadav, V.K., et al., 2022. The state of the art and emerging trends in the wastewater treatment in developing nations. Water. 14(16), 2537. DOI: https://doi.org/10.3390/w14162537
[6] Schoengold, K., Zilberman, D., 2007. Chapter 58 The economics of water, irrigation, and development. Handbook of Agricultural Economics. 3, 2933–2977. DOI: https://doi.org/10.1016/S1574-0072(06)03058-1
[7] Wang, X., Zhang, J., Shahid, S., et al., 2014. Adaptation to climate change impacts on water demand. Mitigation and Adaptation Strategies for Global Change. 21(1), 81–99. DOI: https://doi.org/10.1007/s11027-014-9571-6
[8] Oweis, T., Hachum, A., 2006. Water harvesting and supplemental irrigation for improved water productivity of dry farming systems in West Asia and North Africa. Agricultural Water Management. 80(1–3), 57–73. DOI: https://doi.org/10.1016/j.agwat.2005.07.004
[9] Kumar, S., Meena, R.S., Jhariya, M.K., 2020. Resources use efficiency in agriculture. Springer: Singapore. DOI: https://doi.org/10.1007/978-981-15-6953-1
[10] Koluvek, P.K., Tanji, K.K., Trout, T.J., 1993. Overview of soil erosion from irrigation. Journal of Irrigation and Drainage Engineering. 119(6), 929–946. DOI: https://doi.org/10.1061/(ASCE)0733-9437(1993)119:6(929)
[11] Solomon, K.H., Burt, C.M., 1999. Irrigation sagacity: A measure of prudent water use. Irrigation Science. 18(3), 135–140. DOI: https://doi.org/10.1007/s002710050054
[12] Jamali, M., Soufizadeh, S., Yeganeh, B., et al., 2021. A comparative study of irrigation techniques for energy flow and greenhouse gas (GHG) emissions in wheat agroecosystems under contrasting environments in south of Iran. Renewable and Sustainable Energy Reviews. 139, 110704. DOI: https://doi.org/10.1016/j.rser.2021.110704
[13] Magomadov, V.S., 2019. Deep learning and its role in smart agriculture. Journal of Physics: Conference Series. 1399(4), 044109. DOI: https://doi.org/10.1088/1742-6596/1399/4/044109
[14] Understanding Different Types of Artificial Intelligence with Examples [Internet]. Edureka; 2023 [cited 2023 Nov 20]. Available from: https://www.edureka.co/blog/types-of-artificial-intelligence/
[15] Kingston, G.B., Dandy, G.C., Maier, H.R., 2008. Review of artificial intelligence techniques and their applications to hydrological modeling and water resources management. Part 1—imulation. Water Resources Research Progress. Nova Science Publishers: New York, USA. pp. 67–99.
[16] Applied Technologies, 2020. Communications in computer and information science. Springer International Publishing: Switzerland. DOI: https://doi.org/10.1007/978-3-030-42520-3
[17] Blessy, J.A., Kumar, A. (editors), 2021. Smart irrigation system techniques using artificial intelligence and IoT. 2021 Third International Conference on Intelligent Communication Technologies and Virtual Mobile Networks (ICICV); 2021 Feb 04–06; Tirunelveli, India. USA: IEEE. p. 1355–1359.
[18] Anil Chougule, M., Mashalkar, A.S., 2022. A comprehensive review of agriculture irrigation using artificial intelligence for crop production. Computational Intelligence in Manufacturing. 187–200. DOI: https://doi.org/10.1016/b978-0-323-91854-1.00002-9
[19] Guravaiah, K., Raju, S.S. (editors), 2020. e-Agriculture: Irrigation system based on weather forecasting. 2020 IEEE 15th International Conference on Industrial and Information Systems (ICIIS); 2020 Nov 26–28; RUPNAGAR, India. p. 617–622. DOI: https://doi.org/10.1109/ICIIS51140.2020.9342739
[20] Sensor Based Irrigation System: A review [Internet] [cited 2016 Apr 30]. Available from: https://www.researchgate.net/publication/303842913_Sensor_based_Irrigation_System_A_Review
[21] Alibabaei, K., Gaspar, P.D., Lima, T.M., 2021. Crop yield estimation using deep learning based on climate big data and irrigation scheduling. Energies. 14(11), 3004. DOI: https://doi.org/10.3390/en14113004
[22] Shaikh, F.K., Memon, M.A., Mahoto, N.A., et al., 2022. Artificial intelligence best practices in smart agriculture. IEEE Micro. 42(1), 17–24. DOI: https://doi.org/10.1109/mm.2021.3121279
[23] Pattnaik, P.K., Kumar, R., Pal, S., 2020. Internet of things and analytics for agriculture, Volume 2. Studies in Big Data. Springer: Singapore. DOI: https://doi.org/10.1007/978-981-15-0663-5
[24] Patrizi, G., Bartolini, A., Ciani, L., et al., 2022. A virtual soil moisture sensor for smart farming using deep learning. IEEE Transactions on Instrumentation and Measurement. 71, 1–11. DOI: https://doi.org/10.1109/tim.2022.3196446
[25] Dubois, A., Teytaud, F., Verel, S., 2021. Short term soil moisture forecasts for potato crop farming: A machine learning approach. Computers and Electronics in Agriculture. 180, 105902. DOI: https://doi.org/10.1016/j.compag.2020.105902
[26] Canillas, E.C., Salokhe, V.M., 2002. A decision support system for compaction assessment in agricultural soils. Soil Tillage Research. 65(2), 221–230. DOI: https://doi.org/10.1016/S0167-1987(02)00002-8
[27] De la Rosa, D., Mayol, F., Diaz-Pereira, E., et al., 2004. A land evaluation decision support system (MicroLEIS DSS) for agricultural soil protection. Environmental Modelling & Software. 19(10), 929–942. DOI: https://doi.org/10.1016/j.envsoft.2003.10.006
[28] Nguyen, T.T., Hoang, T.D., Pham, M.T., et al., 2020. Monitoring agriculture areas with satellite images and deep learning. Applied Soft Computing. 95, 106565. DOI: https://doi.org/10.1016/j.asoc.2020.106565
[29] Zamani, A.S., Anand, L., Rane, K.P., et al., 2022. Performance of machine learning and image processing in plant leaf disease detection. Journal of Food Quality. 1–7. DOI: https://doi.org/10.1155/2022/1598796
[30] Virnodkar, S.S., Pachghare, V.K., Patil, V.C., et al., 2020. Remote sensing and machine learning for crop water stress determination in various crops: A critical review. Precision Agriculture. 21(5), 1121–1155. DOI: https://doi.org/10.1007/s11119-020-09711-9
[31] Harakannanavar, S.S., Rudagi, J.M., Puranikmath, V.I., et al., 2022. Plant leaf disease detection using computer vision and machine learning algorithms. Global Transitions Proceedings. 3(1), 305–310. DOI: https://doi.org/10.1016/j.gltp.2022.03.016
[32] Gao, Z., Luo, Z., Zhang, W., et al., 2020. Deep learning application in plant stress imaging: A Review. AgriEngineering. 2(3), 430–446. DOI: https://doi.org/10.3390/agriengineering2030029
[33] Yousaf, A., Kayvanfar, V., Mazzoni, A., et al., 2023. Artificial intelligence-based decision support systems in smart agriculture: Bibliometric analysis for operational insights and future directions. Frontiers in Sustainable Food Systems. 6. DOI: https://doi.org/10.3389/fsufs.2022.1053921
[34] Mohapatra, A.G., Lenka, S.K., 2016. Hybrid decision support system using PLSR-fuzzy model for GSM-based site-specific irrigation notification and control in precision agriculture. International Journal of Intelligent Systems Technologies and Applications. 15(1), 4. DOI: https://doi.org/10.1504/ijista.2016.076101
[35] Pierre, N., Ishimwe Viviane, I.V., Lambert, U., et al., 2023. AI-based real-time weather condition prediction with optimized agricultural resources. European Journal of Technology. 7(2), 36–49. DOI: https://doi.org/10.47672/ejt.1496
[36] Mendes, W.R., Araújo, F.M.U., Dutta, R., et al., 2019. Fuzzy control system for variable rate irrigation using remote sensing. Expert Systems with Applications. 124, 13–24. DOI: https://doi.org/10.1016/j.eswa.2019.01.043
[37] Srivastava, A., Maity, R., 2023. Assessing the potential of AI–ML in urban climate change adaptation and sustainable development. Sustainability. 15(23), 16461. DOI: https://doi.org/10.3390/su152316461
[38] Bruggeman, A. (editor), 2016. The future of sustainable irrigation management in Europe. ENORASIS Conference Abstracts; 27 Nov 2014; IRLA2014 International Symposium; Patras, Greece. p. 1–13.
[39] Bashir, B.A., 2007. Remote sensing derived crop coefficient for estimating crop water requirements for irrigated sorghum in the Gezira Scheme, Sudan. Journal of Environmental Informatics. 10(1), 47–54. DOI: https://doi.org/10.3808/jei.200700099
[40] Bashir, M.A., Hata, T., Tanakamaru, H., et al., 2008. Satellite-based energy balance model to estimate seasonal evapotranspiration for irrigated sorghum: a case study from the Gezira scheme, Sudan. Hydrology and Earth System Sciences. 12(4), 1129–1139. DOI: https://doi.org/10.5194/hess-12-1129-2008
[41] Bwambale, E., Abagale, F.K., Anornu, G.K., 2022. Smart irrigation monitoring and control strategies for improving water use efficiency in precision agriculture: A review. Agricultural Water Management. 260, 107324. DOI: https://doi.org/10.1016/j.agwat.2021.107324
[42] Qazi, S., Khawaja, B.A., Farooq, Q.U., 2022. IoT-Equipped and AI-Enabled next generation smart agriculture: A critical review, current challenges and future trends. IEEE Access. 10, 21219–21235. DOI: https://doi.org/10.1109/access.2022.3152544
[43] Parra, L., Botella-Campos, M., Puerto, H., et al., 2020. Evaluating irrigation efficiency with performance indicators: A case study of citrus in the east of Spain. Agronomy. 10(9), 1359. DOI: https://doi.org/10.3390/agronomy10091359
[44] Obaideen, K., Yousef, B.A.A., AlMallahi, M.N., et al., 2022. An overview of smart irrigation systems using IoT. Energy Nexus. 7, 100124. DOI: https://doi.org/10.1016/j.nexus.2022.100124
Downloads
How to Cite
Issue
Article Type
License
Copyright © 2024 Author(s)

This is an open access article under the Creative Commons Attribution-NonCommercial 4.0 International (CC BY-NC 4.0) License.