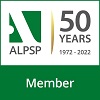
AI Based Urban Resilience Planning: Opportunities and Challenges
DOI:
https://doi.org/10.30564/jees.v6i2.6681Abstract
Urban areas across the globe are confronting escalating flood risks, a crisis exacerbated by the effects of climate change, which necessitates precise hyper-localized risk assessments. This research introduces the application of the AI for resilient cities model for flood risk assessment, focusing specifically on the vulnerable area of Penthakata, located in the coastal city of Puri, Odisha. The hyper-local evaluation of associated flood risks is carried out, particularly at the building level. Leveraging cutting-edge geospatial technology, deep learning methodologies, and multi-parameter analysis, this study offers valuable insights into the flood vulnerabilities in the region. Additionally, the research emphasizes the integration of technology with community volunteers and local knowledge, highlighting the essential role of grassroots-level efforts in effective disaster management. The model is one of its kind combining advanced AI technology with community engagement, the study contributes to a holistic and localized approach to strengthen adaptive capacity in the face of increasing flood risks. The findings offer a compelling case for the adoption of hyperlocal risk assessments for urban and rural areas to be better informed of flood risks, prepared for potential disasters, and implement more effective mitigative measures. Ultimately, it aims to safeguard the lives and livelihoods of vulnerable communities of various regions, offering a model for spatial environmental and community resilience.
Keywords:
Flood risk; Community; Hyperlocal assessment; Climate change; Disaster; Artificial IntelligenceReferences
[1] Tsakiris, G., 2014. Flood risk assessment: concepts, modelling, applications. Natural Hazards and Earth System Sciences. 14(5), 1361–1369.
[2] NDMA. Floods. National Disaster Management Authority; 2023 [cited 2024 May 8]. Available from: https://ndma.gov.in/Natural-Hazards/Floods
[3] Luo, P., Luo, M., Li, F., et al., 2022. Urban flood numerical simulation: Research, methods and future perspectives. Environmental Modelling & Software. 156, 105478. DOI: https://doi.org/10.1016/j.envsoft.2022.105478
[4] Rahman, M., Ningsheng, C., Mahmud, G.I., et al., 2021. Flooding and its relationship with land cover change, population growth, and road density. Geoscience Frontiers. 12(6), 101224. DOI: https://doi.org/10.1016/j.gsf.2021.101224
[5] Mona Hemmati, B.R., Mahmoud, H.N., 2020. The role of urban growth in resilience of communities under flood risk. Earth's Future. 8(3), e2019EF001382. DOI: https://doi.org/10.1029/2019EF001382
[6] de Moel, H., Jongman, B., Kreibich, H., et al., 2015. Flood risk assessments at different spatial scales. Mitigation and Adaptation Strategies for Global Change. 20, 865–890. DOI: https://doi.org/10.1007/s11027-015-9654-z
[7] Wang, L., Cui, S., Li, Y., et al., 2022. A review of the flood management: from flood control to flood resilience. Heliyon. 8(11). DOI: https://doi.org/10.1016/j.heliyon.2022.e11763
[8] Pham, B.T., Luu, C., Dao, D.V., et al., 2021. Flood risk assessment using deep learning integrated with multi-criteria decision analysis. Knowledge-Based Systems. 219. DOI: https://doi.org/10.1016/j.knosys.2021.106899
[9] Ozturk, D., Batuk, F., 2011. Implementataion of GIS-based multicriteria decision analysis with VB in ArcGIS VB. International Journal of Information Technology & Decision Making. 10(6), 1023–1042.
[10] Ghaffarian, S., Taghikhah, F.R., Maier, H.R., 2023. Explainable artificial intelligence in disaster risk management: Achievements and prospective futures. International Journal of Disaster Risk Reduction. 98, 104123. DOI: https://doi.org/10.1016/j.ijdrr.2023.104123
[11] Tripathi, P., 2015. Flood disaster in India: An analysis of trend and preparedness. Interdisciplinary Journal of Contemporary Research. 2.
[12] Mohanty, M.P., Mudgil, S., Karmakar, S., 2020. Flood management in India: A focussed review on the current status and future challenges. International Journal of Disaster Risk Reduction. 49. DOI: https://doi.org/10.1016/j.ijdrr.2020.101660
[13] Mohapatra, S., Harish, V., Dwivedi, G., 2022. Climate change, cyclone and rural communities: Understanding people's perceptions and adaptations in rural eastern India. Materials Today: Proceedings. 49, 412–417. DOI: https://doi.org/10.1016/j.matpr.2021.02.384
[14] Falga, R., Wang, C., 2022. The rise of Indian summer monsoon precipitation extremes and its correlation with long-term changes of climate and anthropogenic factors. Scientific Reports. 12. DOI: https://doi.org/10.1038/s41598-022-16240-0
[15] Ivić, M., 2019. Artificial intelligence and geospatial analysis in disaster management. The International Archives of the Photogrammetry, Remote Sensing and Spatial Information Sciences/International Archives of the Photogrammetry, Remote Sensing and Spatial Information Sciences. XLII-3/W8, 161–166. DOI: https://doi.org/10.5194/isprs-archives-xlii-3-w8-161-2019
[16] Katoch, N., 2023. Resilient urban planning and disaster preparedness for sustainable cities and SDGs: A study of Himachal Pradesh (HP). International Journal of Current Science. 13(3), 102–107.
[17] Panda, S., Mishra, S.P., 2020. Confronting and coping with resilient environment by fishermen community of Penthakata, Puri during Fani. Adalya Journal. 9(1), 230–242.
[18] Aldardasawi, A.F., Eren, B., 2021. Floods and their impact on the environment. Academic Perspective Procedia. 4(2), 42–49. DOI: https://doi.org/10.33793/acperpro.04.02.24
[19] Demir, G., Akyurek, Z., 2016. The importance of precise Digital Elevation Models (DEM) in modelling floods. EGU General Assembly 2016. 18. Available from: https://meetingorganizer.copernicus.org/EGU2016/EGU2016-9604.pdf
[20] Rahman, M., Ningsheng, C., Mahmud, G.I., et al., 2021. Flooding and its relationship with land cover change, population growth, and road density. Geoscience Frontiers. 12(6). DOI: https://doi.org/10.1016/j.gsf.2021.101224
[21] Floods. World Health Organization; 2021 [cited 2024 May 8]. Available from: https://www.who.int/health-topics/floods#tab=tab_1
[22] Jonkman, S.N., Curran, A., Bouwer, L.M., 2024. Floods have become less deadly: An analysis of global flood fatalities 1975–2022. Natural Hazards. 120, 6327–6342. DOI: https://doi.org/10.1007/s11069-024-06444-0
[23] Ali, H., Modi, P., Mishra, V., 2019. Increased flood risk in Indian sub-continent under the warming climate. Weather and Climate Extremes. 25, 100212. DOI: https://doi.org/10.1016/j.wace.2019.100212
[24] Buta, C., Mihai, G., Stănescu, M., 2020. Flood risk assessment based on flood hazard and vulnerability indexes. Ovidius University Annals of Constanta—Series Civil Engineering. 22(1), 127–137. DOI: https://doi.org/10.2478/ouacsce-2020-0014
[25] Chen, W., Wang, W., Huang, G., et al., 2021. The capacity of grey infrastructure in urban flood management: A comprehensive analysis of grey infrastructure and the green-grey approach. International Journal of Disaster Risk Reduction. 54, 102045. DOI: https://doi.org/10.1016/j.ijdrr.2021.102045
[26] [26] Samanta, S., Pal, D.K., Palsamanta, B., 2018. Flood susceptibility analysis through remote sensing, GIS and frequency ratio model. Applied Water Science. 8. DOI: https://doi.org/10.1007/s13201-018-0710-1
[27] Priscillia, S., Schillaci, C., Lipani, A., 2022. Flood susceptibility assessment using artificial neural networks in Indonesia. Artificial Intelligence in Geosciences. 2, 215–222. DOI: https://doi.org/10.1016/j.aiig.2022.03.002
[28] Dhiman, R., VishnuRadhan, R., Eldho, T.I., et al., 2019. Flood risk and adaptation in Indian coastal cities: recent scenarios. Applied Water Science. 9. DOI: https://doi.org/10.1007/s13201-018-0881-9
[29] Das, S., Gupta, A., 2021. Multi-criteria decision based geospatial mapping of flood susceptibility and temporal hydro-geomorphic changes in the Subarnarekha basin, India. Geoscience Frontiers. 12(5), 101206. DOI: https://doi.org/10.1016/j.gsf.2021.101206
[30] Beven, K.K., 1979. A physically based, variable contributing area model of basin hydrology. Hydrological Sciences Bulletin. 24.
[31] Grabs, T., Seibert, J., Bishop, K., et al., 2009. Modeling spatial patterns of saturated areas: A comparison of the topographic wetness index and a dynamic distributed model. Journal of Hydrology. 373(1–2), 15–23. DOI: https://doi.org/10.1016/j.jhydrol.2009.03.031
[32] O' Callaghan, J.F., Mark, D.M., 1984. December. The extraction of drainage networks from digital elevation data. Computer Vision, Graphics, and Image Processing. 28(3), 323–344. DOI: https://doi.org/10.1016/S0734-189X(84)80011-0
[33] Gandhi, G.M., Parthiban, S., Thummalu, N., et al., 2015. Ndvi: Vegetation change detection using remote sensing and GIS—a case study of vellore district. Procedia Computer Science. 57, 1199–1210. DOI: https://doi.org/10.1016/j.procs.2015.07.415
[34] Arnold, C.L., Gibbons, C.J., 2007. Impervious surface coverage: the emergence of a key environmental indicator. Journal of the American Planning Association. 62(2), 243–258. DOI: https://doi.org/10.1080/01944369608975688
[35] Nasir, M., Sederholm, T., Sharma, A., 2022. Dwelling type classification for disaster risk assessment using satellite imagery. 36th Conference on Neural Information Processing Systems (NeurIPS 2022), AI+HADR Workshop. Available from: https://arxiv.org/pdf/2211.11636
[36] Turner, B.L., Lambin, E.F., Reenberg, A., 2007. The emergence of land change science for global environmental change and sustainability. Proceedings of the National Academy of Sciences. 104(52), 20666–20671. DOI: https://doi.org/10.1073/pnas.0704119104
[37] Saaty, T.L., 1980. The analytic hierarchy process: Planning, priority setting, resources allocation. McGraw Hill Higher Education: New York, USA.
[38] Taherdoost, H., 2017. Decision making using the analytic hierarchy process (AHP); A step by step approach. International Journal of Economics and Management Systems. 2.
Downloads
How to Cite
Issue
Article Type
License
Copyright © 2024 Author(s)

This is an open access article under the Creative Commons Attribution-NonCommercial 4.0 International (CC BY-NC 4.0) License.