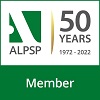
Machine Learning Approach for Short- and Long-Term Global Solar Irradiance Prediction
DOI:
https://doi.org/10.30564/jees.v7i1.7060Abstract
Solar radiation data forecasting algorithms are important, especially in developing countries, as vast solar power plants cannot measure reliable and constant solar irradiance. The challenges of solar irradiance prediction may be resolved by machine learning using weather datasets. This study emphasises the daily and monthly global solar radiation data predictions of three locations, Pretoria, Bloemfontein, and Vuwani, at different provinces in South Africa with various solar radiation distributions. The study evaluated five different machine learning models. Forecasting models were established to evaluate global solar radiation, focusing on input data. The selected forecast models are centered on their ability to perform with time series data. These models use five years of data from meteorological parameters, such as global horizontal irradiance (GHI), relative humidity, wind speed and ambient temperature between 1 January 2018 and 31 December 2022. The datasets from these meteorological parameters are utilised for training and testing the employed algorithms, which are examined using five statistical metrics. Moreover, the inconsistency of the solar irradiance time series was equally assessed using the clearness index. The results from this study demonstrate that the R2 value recording 0.866 datasets in Bloemfontein of random forest algorithm presents the highest performance during the training processes for all models studied, while the random tree in Vuwani showed the lowest performance of R2 of 0.210 with other algorithms in testing processes. Additionally, the maximum solar radiation was found in December for both Pretoria and Bloemfontein, recorded as 5.347 and 5.844 kWh/m2/day, respectively, while it was 4.692 kWh/m2/day at Vuwani in January. Similarly, the average clearness index of 0.605, 0.657 and 0.533 are obtained at Pretoria, Bloemfontein, and Vuwani, respectively. Among the three sites under study, the solar radiation and clearness index are higher in Bloemfontein. Therefore, the proposed algorithms could be used conveniently for short- and long-term solar power plants in South Africa.
Keywords:
Machine Learning; Solar Radiation; Short and Long-Term; Forecasting; Statistical MetricsReferences
[1] Jebli, I., Belouadha, F.-Z., Kabbaj, M.I., et al., 2021. Deep learning based models for solar energy prediction. Advances in Science, Technology and Engineering Systems Journal. 6(1), 349–355.
[2] Apeh, O.O., Meyer, E.L., Overen, O.K., 2022. Contributions of Solar Photovoltaic Systems to Environmental and Socioeconomic Aspects of National Development—A Review. Energies. 15(16), 5963.
[3] Ritchie, H., Roser, M., Rosado, P., 2020. CO₂ and greenhouse gas emissions. Our World in Data.
[4] Okampo, E.J., Nwulu, N.I., 2020. Optimal energy mix for a reverse osmosis desalination unit considering demand response. Journal of Engineering Design and Technology. 18(5), 1287–1303.
[5] Azizi, N., Yaghoubirad, M., Farajollahi, M., et al., 2023. Deep learning based long-term global solar irradiance and temperature forecasting using time series with multi-step multivariate output. Renewable Energy. 206, 135–147.
[6] Maleki, S.A.M., Hizam, H., Gomes, C., 2017. Estimation of hourly, daily and monthly global solar radiation on inclined surfaces: models re-visited. Energies. 10(134).
[7] da Silva, V.J., da Silva, C.R., Almorox, J., et al., 2016. Temperature-based solar radiation models for use in simulated soybean potential yield. Australian Journal of Crop Science. 10(7), 926–932.
[8] Alfailakawi, M.S., Michailos, S., Ingham, D.B., et al., 2022. Multi-temporal resolution aerosols impacted techno-economic assessment of concentrated solar power in arid regions: Case study of solar power tower in Kuwait. Sustainable Energy Technologies and Assessments. 52, 102324.
[9] Akhter, M.N., Mekhilef, S., Mokhlis, H., et al., 2019. Review on forecasting of photovoltaic power generation based on machine learning and metaheuristic techniques. IET Renewable Power Generation. 13(7), 1009–1023.
[10] Antonanzas, J., Osorio, N., Escobar, R., et al., 2016. Review of photovoltaic power forecasting. Solar Energy. 136, 78–111.
[11] Ramedani, Z., Omid, M., Keyhani, A., et al., 2014. Potential of radial basis function based support vector regression for global solar radiation prediction. Renewable and Sustainable Energy Reviews. 39, 1005–1011.
[12] Das, U.K., Tey, K.S., Seyedmahmoudian, M., et al., 2018. Forecasting of photovoltaic power generation and model optimization: A review. Renewable and Sustainable Energy Reviews. 81, 912–928.
[13] Voyant, C., Notton, G., Kalogirou, S., et al., 2017. Machine learning methods for solar radiation forecasting: A review. Renewable Energy. 105, 569–582.
[14] Reikard, G., 2009. Predicting solar radiation at high resolutions: A comparison of time series forecasts. Solar Energy. 83(3), 342–349.
[15] Reikard, G., Haupt, S.E., Jensen, T., 2017. Forecasting ground-level irradiance over short horizons: Time series, meteorological, and time-varying parameter models. Renewable Energy. 112, 474–485.
[16] Diagne, M., David, M., Lauret, P., et al., 2013. Review of solar irradiance forecasting methods and a proposition for small-scale insular grids. Renewable and Sustainable Energy Reviews. 27, 65–76.
[17] Zhang, G., 2003. Time series forecasting using a hybrid ARIMA and neural network model. Neurocomputing. 50, 159–175.
[18] Zhang, G., Patuwo, B.E., Hu, M.Y., 1998. Forecasting with artificial neural networks: The state of the art. International Journal of Forecasting. 14(1), 35–62.
[19] Bayrakçı, H.C., Demircan, C., Keçebaş, A., 2018. The development of empirical models for estimating global solar radiation on horizontal surface: A case study. Renewable and Sustainable Energy Reviews. 81, 2771–2782.
[20] Liu, Y., Zhou, Y., Chen, Y., et al., 2020. Comparison of support vector machine and copula-based nonlinear quantile regression for estimating the daily diffuse solar radiation: A case study in China. Renewable Energy. 146, 1101–1112.
[21] Sharma, A., Kakkar, A., 2018. Forecasting daily global solar irradiance generation using machine learning. Renewable and Sustainable Energy Reviews. 82, 2254–2269.
[22] Mellit, A., Benghanem, M., Arab, A.H., et al., 2005. A simplified model for generating sequences of global solar radiation data for isolated sites: Using artificial neural network and a library of Markov transition matrices approach. Solar Energy. 79(5), 469–482.
[23] Apeh, O.O., Overen, O.K., Meyer, E.L., 2021. Monthly, seasonal and yearly assessments of global solar radiation, clearness index and diffuse fractions in Alice, South Africa. Sustainability. 13(4), 1–15.
[24] Khosravi, A., Koury, R.N.N., Machado, L., et al., 2018. Prediction of hourly solar radiation in Abu Musa Island using machine learning algorithms. Journal of Cleaner Production. 176, 63–75.
[25] VanDeventer, W., Jamei, E., Thirunavukkarasu, G.S., et al., 2019. Short-term PV power forecasting using hybrid GASVM technique. Renewable Energy. 140, 367–379.
[26] Meenal, R., Selvakumar, A.I., 2018. Assessment of SVM, empirical and ANN based solar radiation prediction models with most influencing input parameters. Renewable Energy. 121, 324–343.
[27] Jiang, H., Dong, Y., 2016. A nonlinear support vector machine model with hard penalty function based on glowworm swarm optimization for forecasting daily global solar radiation. Energy Conversion and Management. 126, 991–1002.
[28] Fan, J., Wang, X., Wu, L., et al., 2018. Comparison of Support Vector Machine and Extreme Gradient Boosting for predicting daily global solar radiation using temperature and precipitation in humid subtropical climates: A case study in China. Energy Conversion and Management. 164, 102–111.
[29] Das, U.K., Tey, K.S., Seyedmahmoudian, M., et al., 2017. SVR-based model to forecast PV power generation under different weather conditions. Energies. 10(7), 876.
[30] Wu, Y.-K., Huang, C.-L., Phan, Q.-T., et al., 2022. Completed review of various solar power forecasting techniques considering different viewpoints. Energies. 15(9), 3320.
[31] Kumari, P., Toshniwal, D., 2021. Deep learning models for solar irradiance forecasting: A comprehensive review. Journal of Cleaner Production. 318, 128566.
[32] Yang, D., Ye, Z., Lim, L.H.I., et al., 2015. Very short term irradiance forecasting using the lasso. Solar Energy. 114, 314–326.
[33] Chow, C.W., Urquhart, B., Lave, M., et al., 2011. Intra-hour forecasting with a total sky imager at the UC San Diego solar energy testbed. Solar Energy. 85(11), 2881–2893.
[34] Engerer, N.A., 2015. Minute resolution estimates of the diffuse fraction of global irradiance for southeastern Australia. Solar Energy. 116, 215–237.
[35] Ibrahim, I.A., Khatib, T., 2017. A novel hybrid model for hourly global solar radiation prediction using random forests technique and firefly algorithm. Energy Conversion and Management. 138, 413–425.
[36] Kalogirou, S.A., 2001. Artificial neural networks in renewable energy systems applications: A review. Renewable and Sustainable Energy Reviews. 5(4), 373–401.
[37] Jiang, H., Dong, Y., Xiao, L., 2017. A multi-stage intelligent approach based on an ensemble of two-way interaction model for forecasting the global horizontal radiation of India. Energy Conversion and Management. 137, 142–154.
[38] Olatomiwa, L., Mekhilef, S., Shamshirband, S., et al., 2015. A support vector machine–firefly algorithm-based model for global solar radiation prediction. Solar Energy. 115, 632–644.
[39] Heng, J., Wang, J., Xiao, L., et al., 2017. Research and application of a combined model based on frequent pattern growth algorithm and multi-objective optimization for solar radiation forecasting. Applied Energy. 208, 845–866.
[40] Mishra, A., Kaushika, N.D., Zhang, G., et al., 2008. Artificial neural network model for the estimation of direct solar radiation in the Indian zone. International Journal of Sustainable Energy. 27(3), 95–103.
[41] Olorunfemi, B.O., Nwulu, N.I., Ogbolumani, O.A., 2023. Solar panel surface dirt detection and removal based on Arduino color recognition. MethodsX. 10, 101967.
[42] Yun, G.Y., Kong, H.J., Kim, H., et al., 2012. A field survey of visual comfort and lighting energy consumption in open plan offices. Energy and Buildings. 46, 146–151.
[43] Xuan, Z., Xuehui, Z., Liequan, L., et al., 2019. Forecasting performance comparison of two hybrid machine learning models for cooling load of a large-scale commercial building. Journal of Building Engineering. 21, 64–73.
[44] Runge, J., Zmeureanu, R., Le Cam, M., 2020. Hybrid short-term forecasting of the electric demand of supply fans using machine learning. Journal of Building Engineering. 29, 101144.
[45] Ghodrati, A., Zahedi, R., Ahmadi, A., 2022. Analysis of cold thermal energy storage using phase change materials in freezers. Journal of Energy Storage. 51, 104433.
[46] Fan, C., Xiao, F., Wang, S., 2014. Development of prediction models for next-day building energy consumption and peak power demand using data mining techniques. Applied Energy. 127, 1–10.
[47] Bot, K., Ruano, A., Ruano, M.G., 2020. Forecasting electricity demand in households using MOGA-designed artificial neural networks. IFAC-PapersOnLine. 53(2), 8225–8230.
[48] Bian, H., Zhong, Y., Sun, J., et al., 2020. Study on power consumption load forecast based on K-means clustering and FCM–BP model. Energy Reports. 6, 693–700.
[49] Amasyali, K., El-Gohary, N.M., 2018. A review of data-driven building energy consumption prediction studies. Renewable and Sustainable Energy Reviews. 81, 1192–1205.
[50] Deb, C., Zhang, F., Yang, J., et al., 2017. A review on time series forecasting techniques for building energy consumption. Renewable and Sustainable Energy Reviews. 74, 902–924.
[51] Walker, S., Khan, W., Katic, K., et al., 2020. Accuracy of different machine learning algorithms and added-value of predicting aggregated-level energy performance of commercial buildings. Energy and Buildings. 209, 109705.
[52] Grimaldo, A.I., Novak, J., 2020. Combining machine learning with visual analytics for explainable forecasting of energy demand in prosumer scenarios. Procedia Computer Science. 175, 525–532.
[53] Soulouknga, M.H., Coban, H.H., Falama, R.Z., et al., 2022. Comparison of different models to estimate global solar irradiation in the Sudanese Zone of Chad. Journal of Electronics and Telecommunications. 22(2), 63–71.
[54] Haq, E.U., Lyu, X., Jia, Y., et al., 2020. Forecasting household electric appliances consumption and peak demand based on hybrid machine learning approach. Energy Reports. 6, 1099–1105.
[55] Hafeez, G., Alimgeer, K.S., Khan, I., 2020. Electric load forecasting based on deep learning and optimized by heuristic algorithm in smart grid. Applied Energy. 269, 114915.
[56] Khan, A., Chiroma, H., Imran, M., et al., 2020. Forecasting electricity consumption based on machine learning to improve performance: A case study for the organization of petroleum exporting countries (OPEC). Computers and Electrical Engineering. 86, 106737.
[57] Kazemzadeh, M.-R., Amjadian, A., Amraee, T., 2020. A hybrid data mining driven algorithm for long term electric peak load and energy demand forecasting. Energy. 204, 117948.
[58] Nematchoua, M.K., Orosa, J.A., Afaifia, M., 2022. Prediction of daily global solar radiation and air temperature using six machine learning algorithms; a case of 27 European countries. Ecological Informatics. 69, 101643.
[59] Moreno, A., Gilabert, M.A., Martínez, B., 2011. Mapping daily global solar irradiation over Spain: A comparative study of selected approaches. Solar Energy. 85(9), 2072–2084.
[60] Wang, L., Kisi, O., Zounemat-Kermani, M., et al., 2017. Prediction of solar radiation in China using different adaptive neuro‐fuzzy methods and M5 model tree. International Journal of Climatology. 37(3), 1141–1155.
[61] Chen, J.-L., Li, G.-S., Wu, S.-J., 2013. Assessing the potential of support vector machine for estimating daily solar radiation using sunshine duration. Energy Conversion and Management. 75, 311–318.
[62] Badrudeen, T.U., Nwulu, N.I., Gbadamosi, S.L., 2023. Neural network based approach for steady-state stability assessment of power systems. Sustainability. 15(2), 1667.
[63] Govindasamy, T.R., Chetty, N., 2021. Machine learning models to quantify the influence of PM10 aerosol concentration on global solar radiation prediction in South Africa. Clean Engineering and Technology. 2, 100042.
[64] Obiora, C.N., Ali, A., Hasan, A.N., 2020. Forecasting hourly solar irradiance using long short-term memory (LSTM) network. Proceedings of the 2020 11th International Renewable Energy Congress (IREC); Hammamet, Tunisia; 29–31 October 2020. pp. 1–6.
[65] Ozoegwu, C.G., 2019. Artificial neural network forecast of monthly mean daily global solar radiation of selected locations based on time series and month number. Journal of Cleaner Production. 216, 1–13.
[66] Mehdizadeh, S., Behmanesh, J., Khalili, K., 2016. Comparison of artificial intelligence methods and empirical equations to estimate daily solar radiation. Journal of Atmospheric and Solar-Terrestrial Physics. 146, 215–227.
[67] Antonopoulos, V.Z., Papamichail, D.M., Aschonitis, V.G., et al., 2019. Solar radiation estimation methods using ANN and empirical models. Computers and Electronics in Agriculture. 160, 160–167.
[68] Adeala, A.A., Huan, Z., Enweremadu, C.C., 2015. Evaluation of global solar radiation using multiple weather parameters as predictors for South Africa provinces. Thermal Science. 19(suppl. 2), 495–509.
[69] Bugaje, I.M., 2006. Renewable energy for sustainable development in Africa: a review. Renewable and Sustainable Energy Reviews. 10(6), 603–612.
[70] Younes, S., Claywell, R., Muneer, T., 2005. Quality control of solar radiation data: Present status and proposed new approaches. Energy. 30(9), 1533–1549.
[71] Meddage, D.P.P., Ekanayake, I.U., Herath, S., et al., 2022. Predicting bulk average velocity with rigid vegetation in open channels using tree-based machine learning: a novel approach using explainable artificial intelligence. Sensors. 22(12), 4398.
[72] Al-Rousan, N., Al-Najjar, H., Alomari, O., 2021. Assessment of predicting hourly global solar radiation in Jordan based on Rules, Trees, Meta, Lazy and Function prediction methods. Sustainable Energy Technologies and Assessments. 44, 100923.
[73] Ren, Q., Cheng, H., Han, H., 2017. Research on machine learning framework based on random forest algorithm. Proceedings of the International Conference on Advances in Materials, Machinery, Electronics (AMME 2017); Wuhan, China; 25–26 February 2017. Volume 1820, no. 1.
[74] Ho, T.K., 1995. Random decision forests. Proceedings of 3rd International Conference on Document Analysis and Recognition; Montreal, QC, Canada; 14–16 August 1995. Volume 1, pp. 278–282.
[75] Probst, P., Wright, M.N., Boulesteix, A., 2019. Hyperparameters and tuning strategies for random forest. Wiley Interdisciplinary Reviews: Data Mining and Knowledge Discovery. 9(3), e1301.
[76] Díaz-Uriarte, R., Alvarez de Andrés, S., 2006. Gene selection and classification of microarray data using random forest. BMC Bioinformatics. 7, 1–13.
[77] Nadi, A., Moradi, H., 2019. Increasing the views and reducing the depth in random forest. Expert Systems with Applications. 138, 112801.
[78] Gressling, T., 2020. Automated machine learning. Data Science in Chemistry. Springer Publishing, New York, NY, USA.
[79] Nhu, V.-H., Shahabi, H., Nohani, E., et al., 2020. Daily water level prediction of Zrebar Lake (Iran): A comparison between M5P, random forest, random tree and reduced error pruning trees algorithms. ISPRS International Journal of Geo-Information. 9(8), 479.
[80] Petropoulos, F., Apiletti, D., Assimakopoulos, V., et al., 2022. Forecasting: theory and practice. International Journal of Forecasting. 38(3), 705–871.
[81] Khorasanizadeh, H., Mohammadi, K., Goudarzi, N., 2016. Prediction of horizontal diffuse solar radiation using clearness index based empirical models: A case study. International Journal of Hydrogen Energy. 41(47), 21888–21898.
[82] Gupta, R., Yadav, A.K., Jha, S.K., et al., 2023. Long term estimation of global horizontal irradiance using machine learning algorithms. Optik (Stuttgart). 283, 170873.
[83] Sun, H., Gui, D., Yan, B., et al., 2016. Assessing the potential of random forest method for estimating solar radiation using air pollution index. Energy Conversion and Management. 119, 121–129.
[84] Marquez, R., Coimbra, C.F.M., 2011. Forecasting of global and direct solar irradiance using stochastic learning methods, ground experiments and the NWS database. Solar Energy. 85(5), 746–756.
[85] Lefèvre, M., Oumbe, A., Blanc, P., et al., 2013. McClear: A new model estimating downwelling solar radiation at ground level in clear-sky conditions. Atmospheric Measurement Techniques. 6(9), 2403–2418.
[86] Reikard, G., Hansen, C., 2019. Forecasting solar irradiance at short horizons: Frequency and time domain models. Renewable Energy. 135, 1270–1290.
[87] Géron, A., 2022. Hands-on machine learning with Scikit-Learn, Keras, and TensorFlow. O'Reilly Media, Inc.
[88] Smith, C.J., Bright, J.M., Crook, R., 2017. Cloud cover effect of clear-sky index distributions and differences between human and automatic cloud observations. Solar Energy. 144, 10–21.
[89] Weyll, A.L.C., Kitagawa, Y.K.L., Araujo, M.L.S., et al., 2024. Medium-term forecasting of global horizontal solar radiation in Brazil using machine learning-based methods. Energy. 300, 131549.
[90] Apeh, O.O., Nwulu, N.I., 2024. The water-energy-food-ecosystem nexus scenario in Africa: Perspective and policy implementations. Energy Reports. 11, 5947–5962.
[91] Apeh, O.O., Nwulu, N., 2024. The Food‑Energy‑Water Nexus Optimization: A Systematic Literature Review. Research on World Agricultural Economy. 5(4).
[92] Apeh, O.O., Nwulu, N.I., 2024. Unlocking Economic Growth: Harnessing Renewable Energy to Mitigate Load Shedding in Southern Africa. e-Prime-Advances in Electrical Engineering, Electronics and Energy. 100869.
Downloads
How to Cite
Issue
Article Type
License
Copyright © 2025 Oliver O. Apeh, Nnamdi I. Nwulu

This is an open access article under the Creative Commons Attribution-NonCommercial 4.0 International (CC BY-NC 4.0) License.