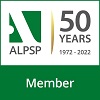
Prediction of Daily Global Solar Radiation on a Horizontal Plane Using Adaptive Neuro-Fuzzy Inference System (ANFIS)
DOI:
https://doi.org/10.30564/jees.v7i1.7079Abstract
In recent years, the world has seen an exponential increase in energy demand, prompting scientists to look for innovative ways to exploit the power sun’s power. Solar energy technologies use the sun's energy and light to provide heating, lighting, hot water, electricity and even cooling for homes, businesses, and industries. Therefore, ground-level solar radiation data is important for these applications. Thus, our work aims to use a mathematical modeling tool to predict solar irradiation. For this purpose, we are interested in the application of the Adaptive Neuro Fuzzy Inference System. Through this type of artificial neural system, 10 models were developed, based on meteorological data such as the Day number (Nj), Ambient temperature (T), Relative Humidity (Hr), Wind speed (WS), Wind direction (WD), Declination (δ), Irradiation outside the atmosphere (Goh), Maximum temperature (Tmax), Minimum temperature (Tmin). These models have been tested by different static indicators to choose the most suitable one for the estimation of the daily global solar radiation. This study led us to choose the M8 model, which takes Nj, T, Hr, δ, Ws, Wd, G0, and S0 as input variables because it presents the best performance either in the learning phase (R² = 0.981, RMSE = 0.107 kW/m², MAE = 0.089 kW/m²) or in the validation phase (R² = 0.979, RMSE = 0.117 kW/m², MAE = 0.101 kW/m²).
Keywords:
Solar Radiation; Adaptive Neuro-Fuzzy Inference System; Prediction Horizontal Plane; Mathematical ModellingReferences
[1] Bahcall, J. , 2001. How the Sun shines. MERCURY-SAN FRANCISCO-. 30(5), 30–37.
[2] Glaser, P.E., 1968. Power from the sun: Its future. Science. 162(3856), 857–861.
[3] Crabtree, G.W., Lewis, N.S., 2008. Solar energy conversion. In: AIP Conference Proceedings. American Institute of Physics. pp. 309–321.
[4] Kropowski, B., Margolis, R., Ton, D., 2009. Harnessing the sun. IEEE Power and Energy Magazine. 7(3), 22–33.
[5] Cameron, A.G.W., 1958. Nuclear astrophysics. Annual review of nuclear science. 8(1), 299–326.
[6] Salpeter, E.E., 1952. Nuclear reactions in the stars. I. Proton-proton chain. Physical review. 88(3), 547.
[7] Salpeter, E.E., 1953. Energy production in stars. Annual review of nuclear science. 2(1), 41–62.
[8] Broggini, C., Bemmerer, D., Guglielmetti, A., et al., 2010. LUNA: Nuclear astrophysics deep underground. Annual Review of Nuclear and Particle Science. 60, 53–73.
[9] Vernazza, J.E., Avrett, E.H., Loeser, R., 1976. Structure of the solar chromosphere. II-The underlying photosphere and temperature-minimum region. Astrophysical Journal Supplement Series. 30, 1–60.
[10] Başkal, S., Kim, Y.S., Noz, M.E., 2019. Einstein's E= mc 2 Derivable from Heisenberg's Uncertainty Relations. Quantum Reports. 1(2), 236–251.
[11] Grevesse, N., Sauval, A.J., 1998. Standard solar composition. Space Science Reviews. 85, 161–174.
[12] Osterbrock, D.E., 1961. The Heating of the Solar Chromosphere, Plages, and Corona by Magnetohydrodynamic Waves. The Astrophysical Journal. 134, 347.
[13] Rozsnyai, B.F., 2001. Solar opacities. Journal of Quantitative Spectroscopy and Radiative Transfer. 71(2–6), 655–663.
[14] El Mghouchi, Y., Ajzoul, T., El Bouardi, A., 2016. Prediction of daily solar radiation intensity by day of the year in twenty-four cities of Morocco. Renewable and Sustainable Energy Reviews. 53, 823–831.
[15] Nfaoui, H., Buret, J., 1993. Estimation of daily and monthly direct, diffuse and global solar radiation in Rabat (Morocco). Renewable Energy. 3(8), 923–930.
[16] Cantoni, R., Rignall, K., 2019. Kingdom of the Sun: a critical, multiscalar analysis of Morocco's solar energy strategy. Energy Research & Social Science. 51, 20–31.
[17] Loutfi, H., Bernatchou, A., Tadili, R., 2017. Generation of horizontal hourly global solar radiation from exogenous variables using an artificial neural network in Fes (Morocco). International Journal of Renewable Energy Research. 7(3), 11.
[18] Berrada, A., Laasmi, M.A., 2021. Technical-economic and socio-political assessment of hydrogen production from solar energy. Journal of Energy Storage. 44, 103448.
[19] Benchrifa, M., Tadili, R., Idrissi, A., et al., 2021. Development of new models for the estimation of hourly components of solar radiation: Tests, comparisons, and application for the generation of a solar database in Morocco. International Journal of Photoenergy. 2021, 1–16.
[20] Benchrifa, M., Essalhi, H., Tadili, R., et al., 2019. Development of a daily databank of solar radiation components for Moroccan territory. International Journal of Photoenergy. 2019.
[21] Benchrifa, M., Mabrouki, J., Essalhi, H., 2023. Estimation of Global Irradiation on Horizontal Plane Using Artificial Neural Network. In: Artificial Intelligence and Smart Environment: ICAISE'2022. Springer International Publishing: Cham, Switzerland. pp. 395–400.
[22] Benchrifa, M., Essalhi, H., Tadili, R., et al., 2022. Estimation of Daily Direct Solar Radiation for Rabat. In: Sustainable Energy Development and Innovation: Selected Papers from the World Renewable Energy Congress (WREC) 2020. Springer International Publishing: Cham, Switzerland. pp. 629–634.
[23] Benchrifa, M., Mabrouki, J., Elouardi, M., et al., 2024. Experimental Study of a New Mixed Solar Dryer Design Case Study: Tomato Drying. In: Technical and Technological Solutions Towards a Sustainable Society and Circular Economy. Springer Nature: Cham, Switzerland. pp. 557–565. DOI: https://doi.org/10.1007/978-3-031-56292-1_44
[24] Benchrifa, M., Mabrouki, J., Elouardi, M., et al., 2024. Studying the Effect of Integration Intelligent Dust Detection and Cleaning System on the Efficiency of Monocrystalline Photovoltaic Panels. In: Technical and Technological Solutions Towards a Sustainable Society and Circular Economy. Springer Nature: Cham, Switzerland. pp. 159–169. DOI: https://doi.org/10.1007/978-3-031-56292-1_12
[25] Benchrifa, M., Mabrouki, J., Ariss, A., et al., 2024. Study of the Physicochemical Properties of Lavender Essential Oil (Lavandula Stoechas). In: Advanced Systems for Environmental Monitoring, IoT and the application of Artificial Intelligence. Springer Nature: Cham, Switzerland. pp. 329–341. DOI: https://doi.org/10.1007/978-3-031-50860-8_20
[26] Qazi, A., Fayaz, H., Wadi, A., et al., 2015. The artificial neural network for solar radiation prediction and designing solar systems: a systematic literature review. Journal of Cleaner Production. 104, 1–12.
[27] Elsherikh, A.H., Sharshir, S.W., Abd Elaziz, M., et al., 2019. Modeling of solar energy systems using artificial neural network: A comprehensive review. Solar Energy. 180, 622–639.
[28] Dorvlo, A.S., Jervase, J.A., Al-Lawati, A., 2002. Solar radiation estimation using artificial neural networks. Applied Energy. 71(4), 307–319.
[29] Mohandes, M., Rehman, S., Halawani, T.O., 1998. Estimation of global solar radiation using artificial neural networks. Renewable energy. 14(1–4), 179–184.
[30] Halabi, L.M., Mekhilef, S., Hossain, M., 2018. Performance evaluation of hybrid adaptive neuro-fuzzy inference system models for predicting monthly global solar radiation. Applied energy. 213, 247–261.
[31] Zou, L., Wang, L., Xia, L., et al., 2017. Prediction and comparison of solar radiation using improved empirical models and Adaptive Neuro-Fuzzy Inference Systems. Renewable energy. 106, 343–353.
[32] Alsharif, M.H., Younes, M.K., 2019. Evaluation and forecasting of solar radiation using time series adaptive neuro‐fuzzy inference system: Seoul city as a case study. IET Renewable Power Generation. 13(10), 1711–1723.
[33] Sumithira, T.R., Nirmal Kumar, A., 2012. Prediction of monthly global solar radiation using adaptive neuro fuzzy inference system (ANFIS) technique over the State of Tamilnadu (India): a comparative study. Applied Solar Energy. 48, 140–145.
[34] Claywell, R., Nadai, L., Felde, I., et al., 2020. Adaptive neuro-fuzzy inference system and a multilayer perceptron model trained with grey wolf optimizer for predicting solar diffuse fraction. Entropy. 22(11), 1192.
[35] Mohanty, S., 2014. ANFIS based prediction of monthly average global solar radiation over Bhubaneswar (State of Odisha). Int. J. Ethics Eng. Manag. Educ. 1(5), 97–101.
[36] Mohammadi, K., Shamshirband, S., Tong, C.W., et al., 2015. Potential of adaptive neuro-fuzzy system for prediction of daily global solar radiation by day of the year. Energy Conversion and Management. 93, 406–413.
[37] Mabrouki, J., Azrou, M., Dhiba, D., et al., 2021. IoT-based data logger for weather monitoring using arduino-based wireless sensor networks with remote graphical application and alerts. Big Data Mining and Analytics. 4(1), 25–32.
[38] Mabrouki, J., Azrou, M., Fattah, G., et al., 2021. Intelligent monitoring system for biogas detection based on the Internet of Things: Mohammedia, Morocco city landfill case. Big Data Mining and Analytics. 4(1), 10–17.
[39] Mabrouki, J., Fattah, G., Al-Jadabi, N., et al., 2021. Study, simulation and modulation of solar thermal domestic hot water production systems. Modeling Earth Systems and Environment. 1–10.
[40] Mabrouki, J., Azrou, M., Farhaoui, Y., et al., 2020. Intelligent system for monitoring and detecting water quality. In: Big Data and Networks Technologies 3. Springer International Publishing. pp. 172–182.
[41] Mabrouki, J., Benchrifa, M., Ennouhi, M., et al., 2023. Geographic Information System for the Study of Water Resources in Chaâba El Hamra, Mohammedia (Morocco). In: Artificial Intelligence and Smart Environment: ICAISE' 2022. Springer International Publishing: Cham. pp. 469–474.
Downloads
How to Cite
Issue
Article Type
License
Copyright © 2025 Hamatti Mohamed, Benchrifa Mohammed, Mohamed Elouardi, Mouhsine Hadine, Mabrouki Jamal, El-Baz Morad, Tadili Rachid

This is an open access article under the Creative Commons Attribution-NonCommercial 4.0 International (CC BY-NC 4.0) License.