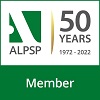
Characterizing Pattern of Topography and Geomorphology in the Hengduan Mountains, Southwest China
DOI:
https://doi.org/10.30564/jees.v7i1.7300Abstract
The Hengduan Mountains, situated on the southeastern edge of the Qinghai-Tibet Plateau, are the longest and widest north-south-oriented mountain range in China, exerting a significant influence on the ecological and geographical pattern. Understanding the topographic and geomorphological characteristics of the Hengduan Mountains is fundamental and crucial for research in related fields such as ecology, geography, and sustainability. In this study, Digital Elevation Model (DEM) data were utilized to extract and analyze the topography and geomorphology (TG) pattern. TG maps have been developed to quantitatively classify the TG types in the Hengduan Mountains by combining the five factors of elevation, slope, aspect, relief and landform. The spatial distribution and quantitative characteristics of these factors were mapped and investigated using geographic information systems. The results revealed that: (1) The Hengduan Mountains exhibit an elongated north-south distribution, with an average elevation of approximately 3746 m, an average slope of around 25°, and an average relief of about 266 m. (2) The Hengduan Mountains display significant elevation differences, with an overall high elevation, characterized by a trend of lower elevation in the east and higher elevation in the west, as well as irregular orientations of various aspects. (3) The 19 landform types were identified; the landform types of the Hengduan Mountains are primarily composed of low-relief high-mountains (42.0618%), low-relief mid-mountains (22.4624%), and high-elevation hills (20.5839%). The results of the study can provide data and information support for the ecology, environmental protection and sustainable development of the Hengduan Mountains.
Keywords:
Topography; Geomorphology; Spatial Pattern; Digital Terrain Analysis; Hengduan MountainsReferences
[1] Szypuła, B., 2017. Hydro-Geomorphology - Models and Trends. Intech Open: Zagreb, Croatia. pp. 1–122.
[2] De Jong, M.G.G., Sterk, H.P., Shinneman, S., et al., 2021. Hierarchical geomorphological mapping in mountainous areas. Journal of Maps. 17, 214–224.
[3] Kazemi Garajeh, M., Feizizadeh, B., Weng, Q., et al., 2022. Desert landform detection and mapping using a semi-automated object-based image analysis approach. Journal of Arid Environments. 199, 104721.
[4] Quesada-Román, A., Peralta-Reyes, M., 2023. Geomorphological mapping global trends and applications. Geographies. 3(3), 610–621.
[5] Bishop, M.P., James, L.A., Shroder, J.F., et al., 2012. Geospatial technologies and digital geomorphological mapping: Concepts, issues and research. Geomorphology. 137, 5–26.
[6] Wang, D., Laffan, S.W., Liu, Y., et al., 2010. Morphometric characterisation of landform from DEMs. International Journal of Geographical Information Science. 24, 305–326.
[7] Saha, S., Paul, G.C., Hembram, T.K., 2019. Classification of terrain based on geo-environmental parameters and their relationship with land use/land cover in Bansloi River basin, Eastern India: RS-GIS approach. Applied Geomatics. 12, 55–71.
[8] Barbălată, L., Comănescu, L., 2021. Environmental sustainability and the inclusion of geomorphosites in tourist activity—case study: The Baiului Mountains, Romania. Sustainability, 13(14), 8094.
[9] Zangana, I., Otto, J.-C., Mäusbacher, R., et al., 2023. Efficient geomorphological mapping based on geographic information systems and remote sensing data: An example from Jena, Germany. Journal of Maps. 19(1), 2172468.
[10] Ferrando, A., Bosino, A., Bonino, E., et al., 2023. Geomorphology and geoheritage in the Piana Crixia Natural Park (NW Italy). Journal of Maps. 19(1), 2257731.
[11] Garcia, G.P.B, Grohmann, C.H., 2019. DEM-based geomorphological mapping and landforms characterization of a tropical karst environment in southeastern. Brazil Journal of South American Earth Sciences. 93, 14–22.
[12] Bollati, I.M., Cavalli, M., 2021. Unraveling the relationship between geomorphodiversity and sediment connectivity in a small alpine catchment. Transactions in GIS. 25(5), 2481–2500.
[13] Coratza, P., Bollati, I.M., Panizza, V., et al., 2021. Advances in geoheritage mapping: Application to iconic geomorphological examples from the Italian landscape. Sustainability. 13(20), 11538.
[14] Na, J., Ding, H., Zhao, W., et al., 2021. Object‐based large‐scale terrain classification combined with segmentation optimization and terrain features: A case study in China. Transactions in GIS. 25, 2939–2962.
[15] Bufalini, M., Materazzi, M., De Amicis, M., et al., 2021. From traditional to modern ‘full coverage' geomorphological mapping: A study case in the Chienti river basin (Marche region, central Italy). Journal of Maps. 17, 17–28.
[16] Ngunjiri, M.W., Libohova, Z., Owens, P.R., et al., 2020. Landform pattern recognition and classification for predicting soil types of the Uasin Gishu Plateau, Kenya Catena. CATENA. 188, 104390.
[17] Brigham, C.A.P., Crider, J.G., 2022. A new metric for morphologic variability using landform shape classification via supervised machine learning. Geomorphology. 399, 108065.
[18] Sărășan, A., Józsa, E., Ardelean, A.C., et al., 2018. Sensitivity of geomorphons to mapping specific landforms from a digital elevation model: A case study of drumlins. Area. 51, 257–267.
[19] Yang, X., Tang, G., Meng, X., et al., 2019. Classification of karst Fenglin and Fengcong landform units based on spatial relations of terrain feature points from DEMs. Remote Sensing. 11(16), 1950.
[20] Xu, Y., Zhu, H., Hu, C., et al., 2021. Deep learning of DEM image texture for landform classification in the Shandong area, China. Frontiers of Earth Science. 16, 352–367.
[21] Li, S., Xiong, L., Tang, G., et al., 2020. Deep learning-based approach for landform classification from integrated data sources of digital elevation model and imagery. Geomorphology. 354, 107045.
[22] Wang, Y., Dai, E., Yin, L., et al., 2018. Land use/land cover change and the effects on ecosystem services in the Hengduan Mountain region, China. Ecosystem Services. 34, 55–67.
[23] Chen, T., Peng, L., Wang, Q., et al., 2017. Measuring the coordinated development of ecological and economic systems in Hengduan Mountain area. Sustainability. 9(8), 1270.
[24] Janowski, L., Tylmann, K., Trzcinska, K., et al., 2022. Exploration of glacial landforms by object-based image analysis and spectral parameters of digital elevation model. IEEE Transactions on Geoscience and Remote Sensing. 60, 1–17.
[25] Zhang, B., Fan, Z., Du, Z., et al., 2020. A geomorphological regionalization using the upscaled DEM: the Beijing-Tianjin-Hebei area, China case study. Scientific Reports. 10, 10532.
[26] Montgomery, D.R., Brandon, M.T., 2002. Topographic controls on erosion rates in tectonically active mountain ranges. Earth and Planetary Science Letters. 201, 481–489.
[27] Xiong, L., Tang, G., Yang, X., et al., 2021. Geomorphology-oriented digital terrain analysis: Progress and perspectives. Journal of Geographical Sciences. 31, 456–476.
[28] Shirvani, A., 2015 Change point analysis of mean annual air temperature in Iran Atmospheric Research. 160, 91–98.
[29] Liu, S., Cui, Y., Lu, F., et al., 2013. The analysis of relief amplitude in Qinghai Province based on GIS. Proceedings of the 21st International Conference on Geoinformatics; Kaifeng, China, 20–22 June 2013. pp. 1–5.
[30] Yang, S.-m., Zhang, Y.-h., Chen, S., 2018. Extraction of terrain relief amplitude based on GIS and change point theory. DEStech Transactions on Computer Science and Engineering. DOI: https://doi.org/10.12783/dtcse/pcmm2018/23712
[31] Cheng, W., Zhou, C., Chai, H., et al., 2010. Quantitative extraction and analysis of basic morphological types of land geomorphology in China. Geo-information Science. 11, 725–736.
[32] Zhu, H., Xu, Y., Cheng, Y., et al., 2019. Landform classification based on optimal texture feature extraction from DEM data in Shandong Hilly Area, China. Frontiers of Earth Science. 13, 641–655.
Downloads
How to Cite
Issue
Article Type
License
Copyright © 2025 Youjun Chen, Yanying Chen, Xiaokang Hu, Jianmeng Feng

This is an open access article under the Creative Commons Attribution-NonCommercial 4.0 International (CC BY-NC 4.0) License.