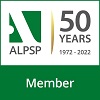
Flood Mapping Methodologies in Google Earth Engine Using Optical and Radar Data: A Comparative Study
DOI:
https://doi.org/10.30564/jees.v7i1.7397Abstract
Floods are among the most severe and frequent natural disasters, impacting numerous countries worldwide. This study investigates flood mapping methodologies utilizing Google Earth Engine (GEE) with Sentinel-1, Sentinel-2, and Landsat data, focusing on the January 2021 Tetouan flood in Morocco. Three approaches were assessed: Sentinel-1 thresholding and NDWI (Normalized Difference Water Index) methods applied to Sentinel-2 and Landsat imagery. The analysis revealed flooded areas of 891 hectares (Sentinel-1), 814 hectares (Sentinel-2), and 1237 hectares (Landsat), validated against ArcGIS (Geographic Information System) results estimating 900 hectares. Sentinel-1 demonstrated superior accuracy with only a 9-hectare deviation and proved effective under cloudy conditions. Sentinel-2 provided a balance between spatial resolution and error levels, with moderate commission and omission errors. Landsat detected the largest flood extent but exhibited a slight overestimation. The study emphasizes the advantages of GEE’s cloud-based platform, which significantly reduced processing time, facilitating rapid flood extent mapping. This scalability and efficiency make GEE an invaluable tool for disaster management. The results underline the potential of these methodologies for accurate and timely flood monitoring, enabling informed decision-making in resilience planning and emergency response. Such advancements are critical for mitigating the impacts of flooding and supporting sustainable disaster management strategies in vulnerable regions worldwide.
Keywords:
Flood; Google Earth Engine; Sentinel-1; Sentinel-2; LandsatReferences
[1] Carmen, F., Abate, N., Faridani, F., et al., 2021. Google earth engine as multi-sensor open-source tool for supporting the preservation of archaeological areas: The case study of flood and fire mapping in Metaponto, Italy. Sensors. 21(5), 1791.
[2] Li, J., Wang, J., Ye, H., 2021. Rapid flood mapping based on remote sensing cloud computing and Sentinel-1. Journal of Physics: Conference Series. 1952, 022051.
[3] Loukili, Y., Lakhrissi, Y., Ali, S.E.B., 2022. Geospatial big data platforms: A comprehensive review. KN-Journal of Cartography and Geographic Information. 72(4), 293−308.
[4] Khan, R., Gilani, H., 2021. Global drought monitoring with big geospatial datasets using Google Earth Engine. Environmental Science and Pollution Research. 28, 17244–17264. DOI: https://doi.org/10.1007/s11356-020-12023-0
[5] Tiwari, V., Kumar, V., Matin, M.A., et al., 2020. Flood inundation mapping-Kerala 2018; Harnessing the power of SAR, automatic threshold detection method and Google Earth Engine. PLoS One. 15(8), e0237324. DOI: https://doi.org/10.1371/journal.pone.0237324
[6] Gumma, M.K., Thenkabail, P.S., Teluguntla, P.G., et al., 2020. Agricultural cropland extent and areas of South Asia derived using Landsat satellite 30-m time-series big-data using random forest machine learning algorithms on the Google Earth Engine cloud. GIScience & Remote Sensing. 57(3), 302–322. DOI: https://doi.org/10.1080/15481603.2019.1690780
[7] Matgen, P., Hostache, R., Schumann, G., et al., 2011. Towards an automated SAR-based flood monitoring system: Lessons learned from two case studies. Physics and Chemistry of the Earth, Parts A/B/C. 36(7–8), 241–252. DOI: https://doi.org/10.1016/j.pce.2010.12.009
[8] Twele, A., Cao, W., Plank, S., et al., 2016. Sentinel-1-based flood mapping: a fully automated processing chain. International Journal of Remote Sensing. 37(13), 2990–3004. DOI: https://doi.org/10.1080/01431161.2016.1192304
[9] Chini, M., Hostache, R., Giustarini, L., et al., 2017. A hierarchical split-based approach for parametric thresholding of SAR images: Flood inundation as a test case. IEEE Transactions on Geoscience and Remote Sensing. 55(12), 6975–6988. DOI: https://doi.org/10.1109/TGRS.2017.2737664
[10] Goffi, A., Stroppiana, D., Brivio, P.A., et al., 2020. Towards an automated approach to map flooded areas from Sentinel-2 MSI data and soft integration of water spectral features. International Journal of Applied Earth Observation and Geoinformation. 84, 101951.
[11] Uddin, K., Matin, M.A., Meyer, F.J., 2019. Operational flood mapping using multi-temporal Sentinel-1 SAR images: A case study from Bangladesh. Remote Sensing. 11(13), 1581.
[12] Devries, B., Huang, C., Armston, J., et al. 2020. Rapid and robust monitoring of flood events using Sentinel-1 and Landsat data on the Google Earth Engine. Remote Sensing of Environment. 240, 111664.
[13] Mehmood, H., Conway, C., Perera, D., 2021. Mapping of flood areas using landsat with google earth engine cloud platform. Atmosphere. 12(7), 866.
[14] Sustainable Development Goals (SDG), 2022. Tangier Tetouan Al Hoceima regional report. Report number 3, December 2022. (in French). Available from: https://www.hcp.ma/region-tanger/Les-Objectifs-de-Developpement-Durable-Rapport-Regional-de-Tanger-Tetouan-Al-Hoceima-Version-francaise_a652.html. (cited 5 November 2024).
[15] Karrouchi, M., Ouazzani, M., Touhami, M., et al., 2016. Mapping of flooding risk areas in the Tangier-Tetouan region: Case of Martil Watershed (Northern Morocco). International Journal of Innovation and Applied Studies. 14, 1019–1035.
[16] Surface Water Detected (SWD), Satellite on January 13, 2021 NE of Tetouan, Province of Tetouan & Prefecture of M'diq-Fnideq, Region of Tanger-Tétouan-Al Hoceïma, Morocco. Available from: https://unosat.org/products/3010 (cited 5 November 2024).
[17] El Gharbaoui, A., 1980. Earth and Man in the Tingitana Peninsula. Study on man and the natural environment in the Western Rif. Works of the Scientific Institute, geology and physical geography series, no. 15. [Geography Thesis]. (in French)
[18] Sentinel-1 Observation Scenario Archive (S1-OSA). Available from: https://sentinel.esa.int/en/web/sentinel/copernicus/sentinel-1/acquisition-plans/observation-scenario-archive (cited 5 November 2024).
[19] Torres, R., Snoeij, P., Geudtner, D., et al., 2012. GMES Sentinel-1 mission. Remote sensing of environment. 120, 9−24.
[20] Baghdadi, N., Bernier, M., Gauthier, R., et al., 2001. Evaluation of C-band SAR data for wetlands mapping. International Journal of Remote Sensing. 22(1), 71−88.
[21] Henry, J.‐B., Chastanet, P., Fellah, K., et al., 2006. Envisat multi‐polarized ASAR data for flood mapping. International Journal of Remote Sensing. 27(10), 1921−1929.
[22] Anusha, N., Bharathi, B., 2019. An overview on Change Detection and a Case Study Using Multi-temporal Satellite Imagery. In Proceedings of the International Conference on Computational Intelligence in Data Science (ICCIDS); Chennai, India, 21−23 February 2019; pp. 1−6. DOI: https://doi.org/10.1109/ICCIDS.2019.8862160
[23] Psomiadis, E., 2016. Flash flood area mapping utilising SENTINEL-1 radar data. In Proceedings of the Earth Resources and Environmental Remote Sensing/GIS Applications VII. SPIE Remote Sensing; Edinburgh, UK, 18 October 2016. pp. 382−392.
[24] Sentinel-1 script. Available from: https://code.earthengine.google.com/1a3f0b3b7fb364d754794c370acec0c9?noload=true (cited 5 November 2024).
[25] GEE API, 2024. Sentinel-1 algorithms. Available from: https://developers.google.com/earth-engine/sentinel1 (cited 5 November 2024).
[26] Vanama, V.S.K., Mandal, D., Rao, Y.S., 2020. GEE4FLOOD: Rapid mapping of flood areas using temporal Sentinel-1 SAR images with Google Earth Engine cloud platform. Journal of Applied Remote Sensing. 14(3), 034505.
[27] Earth Engine Data Catalog Sentinel-2 (EEDC S-2). Available from: https://developers.google.com/earth-engine/datasets/catalog/sentinel-2 (cited 5 November 2024).
[28] Kumar, H., Karwariya, S., Kumar, R., 2022. Google earth engine-based identification of flood extent and flood-affected paddy rice fields using Sentinel-2 MSI and Sentinel-1 SAR data in Bihar state, India. Journal of the Indian Society of Remote Sensing. 50(5), 791−803.
[29] SentiWiki. Available from: https://sentiwiki.copernicus.eu/web/copernicus-programme (cited 5 November 2024).
[30] Sentinel-2 script. Available from: https://code.earthengine.google.com/94df97b38500a21433e7d66fd229b0a1?noload=true (cited 5 November 2024).
[31] Landsat mission. Available from: https://www.usgs.gov/landsat-missions/landsat-satellite-missions (cited 5 November 2024).
[32] Google Cloud Landsat data (GCLD). Available from: https://cloud.google.com/storage/docs/public-datasets/landsat (cited 5 November 2024).
[33] Landsat script. Available from: https://code.earthengine.google.com/2fbf0a6ece18bbfd80e2eb11ab810b7b?noload=true (cited 5 November 2024).
[34] Earth Engine Data Catalog Sentinel-1 (EEDC-S1). Available from: https://developers.google.com/earth-engine/datasets/catalog/COPERNICUS_S1_GRD (cited 5 November 2024).
[35] Lee, J.-S., Jurkevich, L., Dewaele, P., et al., 1994. Speckle filtering of synthetic aperture radar images: A review. Remote Sensing Reviews. 8(4), 313−340.
[36] Abramov, S., Rubel, O., Lukin, V., et al., 2017. Speckle reducing for Sentinel-1 SAR data. In Proceedings of the 2017 IEEE International Geoscience and Remote Sensing Symposium (IGARSS); Fort Worth, TX, USA, 23-28 July 2017. pp. 2353−2356, DOI: https://doi.org/10.1109/IGARSS.2017.8127463
[37] Copernicus Global Land-Cover Layers (CGL-CL). Available from: https://developers.google.com/earth-engine/datasets/catalog/COPERNICUS_Landcover_100m_Proba-V-C3_Global. (cited 5 November 2024).
[38] Main-Knorn, M., Pflug, B., Louis, J., et al., 2017. Sen2Cor for sentinel-2. In Proceedings of the Image and Signal Processing for Remote Sensing XXIII. SPIE Remote Sensing, Warsaw, Poland. Volume 10427, pp. 37−48.
[39] Du, Y., Zhang, Y., Ling, F., et al., 2016. Water bodies' mapping from Sentinel-2 imagery with modified normalized difference water index at 10-m spatial resolution produced by sharpening the SWIR band. Remote Sensing. 8(4), 354.
[40] Li, W., Du, Z., Ling, F., et al., 2013. A comparison of land surface water mapping using the normalized difference water index from TM, ETM+ and ALI. Remote Sensing. 5(11), 5530−5549.
[41] Ortiz, A., Oliver, G., 2006. On the use of the overlapping area matrix for image segmentation evaluation: A survey and new performance measures. Pattern Recognition Letters. 27(16), 1916−1926. DOI: https://doi.org/10.1016/j.patrec.2006.05.002
[42] Van Beers, F., 2018. Using intersection over union loss to improve binary image segmentation [Bachelor's thesis]. University of Groningen: Groningen, The Netherlands.
[43] Boschetti, L., Flasse, S.P., Brivio, P.A., 2004. Analysis of the conflict between omission and commission in low spatial resolution dichotomic thematic products: The Pareto Boundary. Remote Sensing of Environment. 91(3−4), 280−292. DOI: https://doi.org/10.1016/j.rse.2004.02.015
[44] Singha, M., Dong, J., Sarmah, S., et al., 2020. Identifying floods and flood-affected paddy rice fields in Bangladesh based on Sentinel-1 imagery and Google Earth Engine. ISPRS Journal of Photogrammetry and Remote Sensing. 166, 278−293.
[45] Chen, Z., Zhao, S., 2022. Automatic monitoring of surface water dynamics using Sentinel-1 and Sentinel-2 data with Google Earth Engine. International Journal of Applied Earth Observation and Geoinformation. 113, 103010.
Downloads
How to Cite
Issue
Article Type
License
Copyright © 2025 Yassine Loukili, Younes Lakhrissi, Safae Elhaj Ben Ali

This is an open access article under the Creative Commons Attribution-NonCommercial 4.0 International (CC BY-NC 4.0) License.