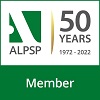
Stochastic Analysis and Modeling of Velocity Observations in Turbulent Flows
DOI:
https://doi.org/10.30564/jees.v6i1.6109Abstract
Highly turbulent water flows, often encountered near human constructions like bridge piers, spillways, and weirs, display intricate dynamics characterized by the formation of eddies and vortices. These formations, varying in sizes and lifespans, significantly influence the distribution of fluid velocities within the flow. Subsequently, the rapid velocity fluctuations in highly turbulent flows lead to elevated shear and normal stress levels. For this reason, to meticulously study these dynamics, more often than not, physical modeling is employed for studying the impact of turbulent flows on the stability and longevity of nearby structures. Despite the effectiveness of physical modeling, various monitoring challenges arise, including flow disruption, the necessity for concurrent gauging at multiple locations, and the duration of measurements. Addressing these challenges, image velocimetry emerges as an ideal method in fluid mechanics, particularly for studying turbulent flows. To account for measurement duration, a probabilistic approach utilizing a probability density function (PDF) is suggested to mitigate uncertainty in estimated average and maximum values. However, it becomes evident that deriving the PDF is not straightforward for all turbulence-induced stresses. In response, this study proposes a novel approach by combining image velocimetry with a stochastic model to provide a generic yet accurate description of flow dynamics in such applications. This integration enables an approach based on the probability of failure, facilitating a more comprehensive analysis of turbulent flows. Such an approach is essential for estimating both short- and long-term stresses on hydraulic constructions under assessment.
Keywords:
Smart modeling; Turbulent flows; Data analysis; Stochastic analysis; Image velocimetryReferences
[1] Dimitriadis, P., Koutsoyiannis, D., Papanicolaou, P., 2016. Stochastic similarities between the microscale of turbulence and hydro-meteorological processes. Hydrological Sciences Journal. 61(9), 1623–1640. DOI: https://doi.org/10.1080/02626667.2015.1085988
[2] Gautam, P., Eldho, T.I., Mazumder, B.S., et al., 2019. Experimental study of flow and turbulence characteristics around simple and complex piers using PIV. Experimental Thermal and Fluid Science. 100, 193–206. DOI: https://doi.org/10.1016/j.expthermflusci.2018.09.010
[3] Mortazavi, M., Le Chenadec, V., Moin, P., et al., 2016. Direct numerical simulation of a turbulent hydraulic jump: Turbulence statistics and air entrainment. Journal of Fluid Mechanics. 797, 60–94. DOI: https://doi.org/10.1017/jfm.2016.230
[4] Leandro, J., Carvalho, R., Chachereau, Y., et al., 2012. Estimating void fraction in a hydraulic jump by measurements of pixel intensity. Experiments in Fluids. 52, 1307–1318. DOI: https://doi.org/10.1007/s00348-011-1257-1
[5] Murzyn, F. (editor), 2010. Assessment of different experimental techniques to investigate the hydraulic jump: do they lead to the same results. 3rd International Junior Researcher and Engineer Workshop on Hydraulic Structures; 2010 May 4–6; Edinburgh, Scotland.
[6] Wang, H., Murzyn, F., 2017. Experimental assessment of characteristic turbulent scales in two-phase flow of hydraulic jump: from bottom to free surface. Environmental Fluid Mechanics. 17, 7–25. DOI: https://doi.org/10.1007/s10652-016-9451-6
[7] Jyoti, J.S., Medeiros, H., Sebo, S., et al., 2023. River velocity measurements using optical flow algorithm and unoccupied aerial vehicles: A case study. Flow Measurement and Instrumentation. 91, 102341. DOI: https://doi.org/10.1016/j.flowmeasinst.2023.102341
[8] Jin, T., Liao, Q., 2019. Application of large scale PIV in river surface turbulence measurements and water depth estimation. Flow Measurement and Instrumentation. 67, 142–152. DOI: https://doi.org/10.1016/j.flowmeasinst.2019.03.001
[9] Emadzadeh, A., Chiew, Y.M. (editors), 2017. Bubble dynamics and PIV measurements in a hydraulic jump. The 37th IAHR World Congress; 2017 Aug 13–18; Kuala Lumpur, Malaysia.
[10] Ayegba, P.O., Edomwonyi‐Otu, L.C., 2020. Turbulence statistics and flow structure in fluid flow using particle image velocimetry technique: A review. Engineering Reports. 2(3), e12138. DOI: https://doi.org/10.1002/eng2.12138
[11] Fujita, I., Muste, M., Kruger, A., 1998. Large-scale particle image velocimetry for flow analysis in hydraulic engineering applications. Journal of hydraulic Research. 36(3), 397–414. DOI: https://doi.org/10.1080/00221689809498626
[12] Fujita, I., Kawamura, Y. (editors), 2001. Discharge measurements of flood flow by imaging technology and float method. Proceedings of the 29th Congress of IAHR; 2001 Sep 16–21; Beijing, China.
[13] Watanabe, K., Fujita, I., Iguchi, M., et al., 2021. Improving accuracy and robustness of space-time image velocimetry (STIV) with deep learning. Water, 13(15), 2079. DOI: https://doi.org/10.3390/w13152079
[14] Shi, R., Leng, X., Chanson, H., 2020. On optical flow techniques applied to breaking surges. Flow Measurement and Instrumentation. 72, 101710. DOI: https://doi.org/10.1016/j.flowmeasinst.2020.101710
[15] Johnson, E.D., Cowen, E.A., 2017. Estimating bed shear stress from remotely measured surface turbulent dissipation fields in open channel flows. Water Resources Research. 53(3), 1982–1996. DOI: https://doi.org/10.1002/2016WR018898
[16] Romagnoli, M., Carvalho, R., Leandro, J., 2013. Turbulence characterization in a gully with reverse flow. Journal of Hydraulic Engineering. 139(7), 736–744. DOI: https://doi.org/10.1061/(ASCE)HY.1943-7900.0000737
[17] Pope, S.B., 2001. Turbulent flows. Measurement Science and Technology. 12(11). DOI: https://doi.org/10.1088/0957-0233/12/11/705
[18] Papoulis, A., 1990. Probability and statistics. Prentice-Hall: New Jersey. pp. 219.
[19] Patnaik, P.B., 1949. The Non-Central χ2- and F-Distribution and their applications. Biometrika. 36 (½), 202–232. DOI: https://doi.org/doi:10.2307/2332542
[20] Nadarajah, S., Pogány, T.K., 2016. On the distribution of the product of correlated normal random variables. Comptes Rendus. Mathématique. 354(2), 201–204. DOI: https://doi.org/10.1016/j.crma.2015.10.019
[21] Cui, G., Yu, X., Iommelli, S., et al., 2016. Exact distribution for the product of two correlated Gaussian random variables. IEEE Signal Processing Letters. 23(11), 1662–1666. DOI: https://doi.org/10.1109/LSP.2016.2614539
[22] Zare, A., Georgiou, T.T., Jovanović, M.R., 2020. Stochastic dynamical modeling of turbulent flows. Annual Review of Control, Robotics, and Autonomous Systems. 3, 195–219. DOI: https://doi.org/10.1146/annurev-control-053018-023843
[23] Czibere, T., 2006. Calculating turbulent flows based on a stochastic model. Journal of Computational and Applied Mechanics. 7(2), 155–188.
[24] Rozos, E., Dimitriadis, P., Mazi, K., et al., 2020. On the uncertainty of the image velocimetry method parameters. Hydrology. 7(3), 65. DOI: https://doi.org/10.3390/hydrology7030065
[25] Rozos, E., Mazi, K., Koussis, A.D., 2021. Probabilistic evaluation and filtering of image velocimetry measurements. Water. 13(16), 2206. DOI: https://doi.org/10.3390/w13162206
[26] Rozos, E., Mazi, K., Lykoudis, S., 2022. On the accuracy of particle image velocimetry with citizen videos—five typical case studies. Hydrology. 9(5), 72. DOI: https://doi.org/10.3390/hydrology9050072
[27] Wijaya, F., Liu, W.C., Huang, W.C., 2023. Comparative assessment of different image velocimetry techniques for measuring river velocities using unmanned aerial vehicle imagery. Water. 15(22), 3941. DOI: https://doi.org/10.3390/w15223941
[28] Koutsoyiannis, D., 1999. Optimal decomposition of covariance matrices for multivariate stochastic models in hydrology. Water Resources Research. 35(4), 1219–1229. DOI: https://doi.org/10.1029/1998WR900093
[29] Matalas, N.C., 1967. Mathematical assessment of synthetic hydrology. Water Resources Research. 3(4), 937–945. DOI: https://doi.org/10.1029/WR003i004p00937
[30] Goodfellow, I., Bengio, Y., Courville, A., 2016. Deep learning. MIT Press: Cambridge. pp. 40.
[31] Rawlings, J.O., Pantula, S.G., Dickey, D.A., 1998. Applied regression analysis: A research tool. Springer: New York. pp. 58.
[32] Searle, S.R., 1982. Matrix algebra useful for statistics. John Wiley & Sons: New York.
[33] Koutsoyiannis, D., 2023. Stochastics of hydroclimatic extremes—A cool look at risk, edition 3. Kallipos Open Academic Editions: Athens. DOI: https://doi.org/10.57713/kallipos-1
[34] Biferale, L., Boffetta, G., Castaing, B., 2003. Fully developed turbulence. The Kolmogorov legacy in physics. Springer-Verlag: Heidelberg. pp. 149–172.
[35] Hyndman, R.J., Fan, Y., 1996. Sample quantiles in statistical packages. The American Statistician. 50(4), 361–365.
[36] Nordin, C.F., McQuivey, R.S., Mejia, J.M., 1972. Hurst phenomenon in turbulence. Water Resources Research. 8(6), 1480–1486. DOI: https://doi.org/10.1029/WR008i006p01480
[37] Dimitriadis, P., Papanicolaou, P. (editors), 2012. Statistical analysis of turbulent positively buoyant jets. European Geosciences Union General Assembly 2012; 2012 Apr 22–27; Vienna, Austria.
[38] Te Chow, V., Maidment, D.R., Mays, L.W., 1988. Applied hydrology. McGraw-Hill: New York.
[39] Kitanidis, P.K., 1993. Geostatistics. The handbook of hydrology. McGraw-Hill: New York.
[40] Lam, R., Sanchez-Gonzalez, A., Willson, M., et al., 2023. Learning skillful medium-range global weather forecasting. Science. 382(6677), 1416–1421. DOI: https://doi.org/10.1126/science.adi2336
Downloads
How to Cite
Issue
Article Type
License
Copyright © 2024 Author(s)

This is an open access article under the Creative Commons Attribution-NonCommercial 4.0 International (CC BY-NC 4.0) License.