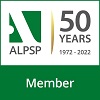
Ensemble Machine Learning Applied to Assessment and Mapping of Low and Moderate Slopes Landslide Susceptibility in Hamam Nbail, Algeria
DOI:
https://doi.org/10.30564/jees.v7i2.7699Abstract
The municipality of Hammam N’bails, located 37 km east of the capital of Guelma province (eastern Algeria), is accessible via RN20 and CW19 roads. It borders the municipalities of Khemissa and El Henancha in Souk-Ahras province. With a population of approximately 16,000 and covering an area of 164 km², this region is characterized by mountainous terrain, with elevations ranging from 112 to 292 meters. The area experiences cold, snowy winters and hot, dry summers, with an average annual rainfall of about 600 mm. Renowned for its natural thermal springs, Hammam N’bails is also a notable tourist destination. The rugged topography of the region leads to frequent landslides, particularly on medium and low slopes. Landslide susceptibility is assessed using raster calculators in ArcGIS and efficient machine learning algorithms, such as Decision Trees, Bagging, Random Forest, SVM, and MLP. Factors considered in the analysis include slope, elevation, geology, aspect, proximity to streams and roads, land cover, and rainfall. The performance of these models is evaluated using ROC-AUC curves, providing a robust method to understand and mitigate geological risks in this area.
Keywords:
Landslide; Low and Middle Slopes; Susceptibility; Machine Learning; Hamam NbailReferences
[1] Djerbal, L., Melbouci, B., 2012. Le glissement de terrain d’Ain El Hammam (Algerie): causes et evolution. Bull Eng Geol Environ. 71(3). Available from: https://search.ebscohost.com/login.aspx?direct=true&profile=ehost&scope=site&authtype=crawler&jrnl=14359529&AN=78312659&h=eM8K%2F60uULXGO639j2P6lt1VevXgptFYtNOX%2B2CvaATW9R6nqX2pIYDH4Y1xDDNP7L%2BaS99VclKRmBorAIOjeg%3D%3D&crl=c (cited 27 October 2024).
[2] Achour, Y., Saidani, Z., Touati, R., et al., 2021. Assessing landslide susceptibility using a machine learning-based approach to achieving land degradation neutrality. Environmental Earth Sciences. 80(17), 575. DOI: https://doi.org/10.1007/s12665-021-09889-9
[3] Kadavi, P.R., Lee, C.W., Lee, S., 2019. Landslide-susceptibility mapping in Gangwon-do, South Korea, using logistic regression and decision tree models. Environmental Earth Sciences. 78(4), 116. DOI: https://doi.org/10.1007/s12665-019-8119-1
[4] Nourani, V., Pradhan, B., Ghaffari, H., et al., 2014. Landslide susceptibility mapping at Zonouz Plain, Iran using genetic programming and comparison with frequency ratio, logistic regression, and artificial neural network models. Nat Hazards. 71(1), 523–547. DOI: https://doi.org/10.1007/s11069-013-0932-3
[5] Nhu, V.H., Shirzadi, A., Shahabi, H., et al., 2020. Shallow landslide susceptibility mapping: A comparison between logistic model tree, logistic regression, naïve bayes tree, artificial neural network, and support vector machine algorithms. International Journal of Environmental Research and Public Health. 17(8), 2749. DOI: https://doi.org/10.3390/ijerph17082749
[6] Akgun, A., 2012. A comparison of landslide susceptibility maps produced by logistic regression, multi-criteria decision, and likelihood ratio methods: a case study at İzmir, Turkey. Landslides. 9(1), 93–106. DOI: https://doi.org/10.1007/s10346-011-0283-7
[7] Akgun, A., Türk, N., 2010. Landslide susceptibility mapping for Ayvalik (Western Turkey) and its vicinity by multicriteria decision analysis. Environmental Earth Sciences. 61(3), 595–611. DOI: https://doi.org/10.1007/s12665-009-0373-1
[8] Lee, S., Hong, S.M., Jung, H.S., 2017. A support vector machine for landslide susceptibility mapping in Gangwon Province, Korea. Sustainability. 9(1), 48. DOI: https://doi.org/10.3390/su9010048
[9] Ohlmacher, G.C., Davis, J.C., 2003. Using multiple logistic regression and GIS technology to predict landslide hazard in northeast Kansas, USA. Engineering Geology. 69(3–4), 331–343. DOI: https://doi.org/10.1016/S0013-7952(03)00069-3
[10] Didier, C., Tritsch, J.J., Watelet, J.M., et al., 1999. Évaluation du risque d’instabilité en surface à l’aplomb d’une ancienne carrière souterraine. Principes d’une analyse par configurations types. In: 9 Congrès International de Mécanique des Roches. pp. 9–14. Available from: https://ineris.hal.science/ineris-00972172/ (cited 27 October 2024).
[11] Pradhan, B., 2010. Application of an advanced fuzzy logic model for landslide susceptibility analysis. International Journal of Computational Intelligence Systems. 3(3), 370. DOI: https://doi.org/10.2991/ijcis.2010.3.3.12
[12] Kavzoglu, T., Sahin, E.K., Colkesen, I., 2014. Landslide susceptibility mapping using GIS-based multi-criteria decision analysis, support vector machines, and logistic regression. Landslides. 11(3), 425–39. DOI: https://doi.org/10.1007/s10346-013-0391-7
[13] Ayalew, L., Yamagishi, H., 2005. The application of GIS-based logistic regression for landslide susceptibility mapping in the Kakuda-Yahiko Mountains, Central Japan. Geomorphology. 65(1–2), 15–31. DOI: https://doi.org/10.1016/j.geomorph.2004.06.010
[14] Ayalew, L., Yamagishi, H., Marui, H., et al., 2005. Landslides in Sado Island of Japan: Part II. GIS-based susceptibility mapping with comparisons of results from two methods and verifications. Engineering Geology. 81(4), 432–445. DOI: https://doi.org/10.1016/j.enggeo.2005.08.004
[15] Achour, Y., Pourghasemi, H.R., 2020. How do machine learning techniques help in increasing accuracy of landslide susceptibility maps? Geoscience Frontiers. 11(3), 871–883. DOI: https://doi.org/10.1016/j.gsf.2019.10.001
[16] Van Westen, C.J., Van Asch, T.W.J., Soeters, R., 2006. Landslide hazard and risk zonation—why is it still so difficult? Bull Eng Geol Environ. 65(2), 167–84. DOI: https://doi.org/10.1007/s10064-005-0023-0
[17] Sharma, R.H., 2013. Evaluating the effect of slope curvature on slope stability by a numerical analysis. Australian Journal of Earth Sciences. 60(2), 283–290. DOI: https://doi.org/10.1080/08120099.2013.762942
[18] Ohlmacher, G.C., 2007. Plan curvature and landslide probability in regions dominated by earth flows and earth slides. Engineering Geology. 91(2–4), 117–134. DOI: https://doi.org/10.1016/j.enggeo.2007.01.005
[19] Keller, E.A., Bean, G., Best, D., 2015. Fluvial geomorphology of a boulder-bed, debris-flow—Dominated channel in an active tectonic environment. Geomorphology. 243, 14–26. DOI: https://doi.org/10.1016/j.geomorph.2015.04.012
[20] Promper, C., Puissant, A., Malet, J.P., et al., 2014. Analysis of land cover changes in the past and the future as contribution to landslide risk scenarios. Applied Geography. 53, 11–19. DOI: https://doi.org/10.1016/j.apgeog.2014.05.020
[21] Matougui, Z., Djerbal, L., Bahar, R., 2023. A comparative study of heterogeneous and homogeneous ensemble approaches for landslide susceptibility assessment in the Djebahia region, Algeria. Environ Sci Pollut Res. 31(28), 40554–40580. DOI: https://doi.org/10.1007/s11356-023-26247-3
[22] Cellek, S., 2021. The effect of aspect on landslide and its relationship with other parameters. In: Landslides. IntechOpen. Available from: https://books.google.com/books?hl=fr&lr=&id=LLGTEAAAQBAJ&oi=fnd&pg=PA13&dq=aspect+and+landslides&ots=GgtlXVrNhN&sig=EqWdKBtrDlcb3HmcKdsMkUb7Iys (cited 27 October 2024).
[23] Çellek, S., 2016. Linear parameters causing landslides: A case study of distance to the road, fault, drainage. Kocaeli Journal of Science and Engineering. 6(2), 94–113. DOI: https://doi.org/10.34088/kojose.1117817
[24] Pham, B.T., Tien, Bui, D., Prakash, I., 2017. Landslide Susceptibility Assessment Using Bagging Ensemble Based Alternating Decision Trees, Logistic Regression and J48 Decision Trees Methods: A Comparative Study. Geotech Geol Eng. 35(6), 2597–611. DOI: https://doi.org/10.1007/s10706-017-0264-2
[25] Dou, J., Yunus, A.P., Bui, D.T., et al., 2020. Improved landslide assessment using support vector machine with bagging, boosting, and stacking ensemble machine learning framework in a mountainous watershed, Japan. Landslides. 17(3), 641–658. DOI: https://doi.org/10.1007/s10346-019-01286-5
[26] Zhao, Y., Zhang, Y., 2008. Comparison of decision tree methods for finding active objects. Advances in Space Research. 41(12), 1955–1959. DOI: https://doi.org/10.1016/j.asr.2007.07.020
[27] Bishop, C.M., 1995. Neural networks for pattern recognition. Oxford University Press. Available from: https://books.google.com/books?hl=fr&lr=&id=T0S0BgAAQBAJ&oi=fnd&pg=PP1&dq=Bishop,+1995&ots=jO5TqJ6Bli&sig=mduOPrqben_hr8wa7lsPFrQ9IyI (cited 27 October 2024).
[28] Goodfellow, I., Bengio, Y., Courville, A., 2016. Deep feedforward networks. Deep Learn. Available from: https://mnassar.github.io/deeplearninghandbook/slides/06_mlp.pdf (cited 27 October 2024).
[29] Cheng, Y.S., Yu, T.T., Son, N.T., 2021. Random forests for landslide prediction in Tsengwen river watershed, central Taiwan. Remote Sensing. 13(2), 199. DOI: https://doi.org/10.3390/rs13020199
[30] Raschka, S., 2014. An Overview of General Performance Metrics of Binary Classifier Systems. arXiv. Available from: http://arxiv.org/abs/1410.5330 (cited 27 October 2024).
[31] Zhang, Z., 2016. Introduction to machine learning: k-nearest neighbors. Annals of Translational Medicine. 4(11), 218. DOI: https://doi.org/10.21037/atm.2016.03.37
[32] Pandey, A., Jain, A., 2017. Comparative analysis of KNN algorithm using various normalization techniques. International Journal of Computer Network and Information Security. 10(11), 36. Available from: https://www.researchgate.net/profile/Achin-Jain-2/publication/320932252_Comparative_Analysis_of_KNN_Algorithm_using_Various_Normalization_Techniques/links/652769a261c4044c404cda3a/Comparative-Analysis-of-KNN-Algorithm-using-Various-Normalization-Techniques.pdf (cited 27 October 2024).
[33] Bell, D.A., 1978. Decision Trees, Tables, and Lattices. In: Batchelor, B.G., ed. Pattern Recognition. Springer US. pp. 117–141. DOI: https://doi.org/10.1007/978-1-4613-4154-3_5
[34] itten, I.H., Frank, E., Hall, M.A., et al., 2005. Practical machine learning tools and techniques. In: Data mining. Elsevier, Amsterdam, The Netherlands. pp. 403–413. Available from: https://sisis.rz.htw-berlin.de/inh2012/12401301.pdf (cited 27 October 2024).
[35] Murthy, S.K., Salsberg, S.L., 1998. Investigations of the greedy heuristic for classification tree induction. Available from: https://citeseerx.ist.psu.edu/document?repid=rep1&type=pdf&doi=e212e4a7609b732125fa011b2476d3e27abb65b8 (cited 27 October 2024).
[36] Breiman, L., Ihaka, R., 1984. Nonlinear discriminant analysis via scaling and ACE. Department of Statistics, University of California Davis One Shields Avenue. Available from: https://citeseerx.ist.psu.edu/document?repid=rep1&type=pdf&doi=ea4bdeb0ba42ed22c85290528372941678680755 (cited 27 October 2024).
[37] Quinlan, J.R., 1987. Generating production rules from decision trees. In: ijcai. Citeseer. pp. 304–307. Available from: https://citeseerx.ist.psu.edu/document?repid=rep1&type=pdf&doi=ea4bdeb0ba42ed22c85290528372941678680755 (cited 27 October 2024).
[38] Hssina, B., Merbouha, A., Ezzikouri, H., et al., 2014. A comparative study of decision tree ID3 and C4.5. Int J Adv Comput Sci Appl. 4(2), 13–19. Available from: https://www.academia.edu/download/34582216/Paper_3-A_comparative_study_of_decision_tree_ID3_and_C4.5.pdf (cited 27 October 2024).
[39] Li, X., Claramunt, C., 2006. A Spatial Entropy-Based Decision Tree for Classification of Geographical Information. Trans GIS. 10(3), 451–467. Available from: https://onlinelibrary.wiley.com/doi/10.1111/j.1467-9671.2006.01006.x (cited 27 October 2024).
Downloads
How to Cite
Issue
Article Type
License
Copyright © 2025 Harfouche Ameur; Djerbal Lynda; Ramdane Bahar

This is an open access article under the Creative Commons Attribution-NonCommercial 4.0 International (CC BY-NC 4.0) License.