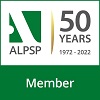
SIF: Satellite Image Fusion for Deforestation Analysis in the Amazon Using S-1 and S-2 Data for LULC Applications
DOI:
https://doi.org/10.30564/jees.v7i6.9190Abstract
Deforestation is the purpose of converting forest into land and reforestation compared to deforestation is very low. That’s why closely and accurately deforestation monitoring using Sentinel-1 and Sentinel-2 satellite images for better vision is required. This paper proposes an effective image fusion technique that combines S-1/2 data to improve the deforested areas. Based on review, Optical and SAR image fusion produces high-resolution images for better deforestation monitoring. To enhance the S-1/2 images, preprocessing is needed as per requirements and then, collocation between the two different types of images to mitigate the image registration problem, and after that, apply an image fusion machine learning approach, PCA-Wavelet. As per analysis, PCA helps to maintain spatial resolution, and Wavelet helps to preserve spectral resolution, gives better-fused images compared to other techniques. As per results, 2019 S-2 preprocessed collocated image enhances 42.2508 km2 deforested area, S-1 preprocessed collocated image enhances 23.7918 km2 deforested area, and after fusion of the 2019 S-1/2 images, it enhances 16.5335 km2 deforested area. Similarly, the 2023 S-2 preprocessed collocated image enhances 49.2216 km2 deforested area, S-1 preprocessed collocated image enhances 23.8459 km2 deforested area after fusion of the 2023 S-1/2 images, enhancing 35.9185 km2 deforested area. These improvements show that combining data sources gives a clearer and more reliable picture of forest loss over time. The overall paper objective is to apply effective techniques for image fusion of Brazil's Amazon Forest and analyze the difference between collocated image pixels and fused image pixels for accurate analysis of deforested area.
Keywords:
Amazon Deforestation; Sentinel-1; Sentinel-2; Collocation Band Math; PCA-WaveletReferences
[1] Sesha Sai, M.V.R., Ramana, K.V., Hebbar, R., et al., Remote Sensing Applications. National Remote Sensing Centre: Hyderabad, Telangana, India. Available from https://bhuvan.nrsc.gov.in/bhuvan/PDF/e-book/Chap_7_Geosci.pdf (1 August 2024 ).
[2] Chen, J., Chen, J., Liao, A., et al., 2015. Global land cover mapping at 30 m resolution: A POK-based operational approach. ISPRS Journal of Photogrammetry and Remote Sensing. 103, 7-27. DOI: https://doi.org/10.1016/j.isprsjprs.2014.09.002
[3] Hansen, M.C., Potapov, P.V., Moore, R., et al., 2013. High-Resolution Global Maps of 21st-Century Forest Cover Change. Science. 342(6160), 850–853. DOI: https://doi.org/10.1126/science.1244693
[4] TAQUARY, Evandro Carrijo. Deep learning for accurate identification of deforestation using high-resolution satellite images. 2019. 64 p. Dissertation (Master's in Computer Science) - Federal University of Goiás, Goiânia, 2019.
[5] INPE, 2020. National Institute for Space Research - Annual Deforestation in Brazilian Savannah. Available from: http://www.obt.inpe.br/cerrado ( 1 August 2024 ).
[6] Liu, B., Pan, L., Qi, Y., et al., 2021. Land Use and Land Cover Change in the Yellow River Basin from 1980 to 2015 and Its Impact on the Ecosystem Services. Land. 10(10), 1080. Doi: https://doi.org/10.3390/land10101080
[7] Watson, R.T., Noble, I.R., Bolin, B., et al., 2000. Land Use, Land-Use Change, and Forestry. Cambridge University Press: Cambridge, UK.
[8] Grekousis, G., Mountrakis, G., Kavouras, M., 2015. An overview of 21 global and 43 regional land cover mapping products. International Journal of Remote Sensing. 36(21), 5309–5335. DOI: https://doi.org/10.1080/01431161.2015.1093195
[9] Curtis, P.G., Slay, C.M., Harris, N.L., et al., 2018. Classifying drivers of global forest loss. Science. 361(6407), 1108–1111. DOI: https://doi.org/10.1126/science.aau3445
[10] Torres, R., Snoeij, P., Geudtner, D., et al., 2012. GMES Sentinel-1 mission. Remote Sensing of Environment. 120, 9–24. DOI: https://doi.org/10.1016/j.rse.2011.05.028
[11] Reiche, J., Hamunyela, E., Verbesselt, J., Hoekman, D., & Herold, M. (2018). Improving near-real time deforestation monitoring in tropical dry forests by combining dense Sentinel-1 time series with Landsat and ALOS-2 PALSAR-2. Remote Sensing of Environment, 204, 147-161.[12] Drusch, M., Del Bello, U., Carlier, S., et al., 2012. Sentinel-2: ESA's Optical High-Resolution Mission for GMES Operational Services. Remote Sensing of Environment. 120, 25–36. DOI: https://doi.org/10.1016/j.rse.2011.11.026
[12] Immitzer, M., Atzberger, C., Koukal, T., 2012. Tree species classification with random forest using very high spatial resolution 8-band WorldView-2 satellite data. Remote sensing. 4(9), 2661–2693. DOI: https://doi.org/10.3390/rs4092661
[13] Dagne, S.S., Hirpha, H.H., Tekoye, A.T. et al. Fusion of sentinel-1 SAR and sentinel-2 MSI data for accurate Urban land use-land cover classification in Gondar City, Ethiopia. Environ Syst Res 12, 40 (2023). https://doi.org/10.1186/s40068-023-00324-5
[14] Zhang, J., 2010. Multi-source remote sensing data fusion: status and trends. International Journal of Image and Data Fusion. 1(1), 5–24. https://doi.org/10.1080/19479830903561035
[15] Pandey, P. C., & Arellano, P. (Eds.). (2022). Advances in remote sensing for forest monitoring. John Wiley & Sons.
[16] FAO, 2020. Global Forest Resources Assessment 2020: Main report. Food and Agriculture Organization of the United Nations: Rome, Italy.
[17] Kader, M.A., Jahan, I., 2019. A review of the application of remote sensing technologies in earthquake disaster management: Potentialities and challenges. Proceedings of the International Conference on Disaster Risk Management; 12–14 January 2019; Dhaka, Bangladesh. pp. 12–14.
[18] Sommervold, O., Gazzea, M., Arghandeh, R., 2023. A survey on SAR and optical satellite image registration. Remote Sensing. 15(3), 850. DOI: https://doi.org/10.3390/rs15030850
[19] Saatchi, S., 2019. SAR Methods for Mapping and Monitoring Forest Biomass. In: Flores, A., Herndon, K., Thapa, R., et al. (eds.). SAR Handbook: Comprehensive Methodologies for Forest Monitoring and Biomass Estimation. NASA: Washington, DC, USA. pp. 207–246.
[20] Lopes, M., Frison, P.-L., Crowson, M., et al., 2020. Improving the accuracy of land cover classification in cloudpersistent areas using optical and radar satellite image timeseries. Methods in Ecology and Evolution. 11(4), 532–541. DOI: https://doi.org/10.1111/2041-210X.13359
[21] Heckel, K., Urban, M., Schratz, P., et al., 2020. Predicting forest cover in distinct ecosystems: The potential of multi-source Sentinel-1 and-2 data fusion. Remote Sensing. 12(2), 302. DOI: https://doi.org/10.3390/rs12020302
[22] Liu, X., Hu, G., Chen, Y., et al., 2019. High-resolution multi-temporal mapping of global urban land using Landsat images based on the Google Earth Engine Platform. Remote Sensing of Environment. 209, 227–239.
[23] Berger, M., Moreno, J., Johannessen, J.A., et al., 2012. ESA's sentinel missions in support of Earth system science. Remote sensing of environment. 120, 84–90.
[24] Abdikan, S., Sanli, F.B., Ustuner, M., et al., 2016. Land cover mapping using sentinel-1 SAR data. The International Archives of the Photogrammetry, Remote Sensing and Spatial Information Sciences. 41, 757–761. DOI: https://doi.org/10.5194/isprs-archives-XLI-B7-757-2016
[25] Torres, R., Navas-Traver, I., Bibby, D., et al., 2017. Sentinel-1 SAR system and mission. Proceedings of the 2017 IEEE Radar Conference (RadarConf); 8–12 May 2017; Seattle, WA, USA. pp. 1582–1585.
[26] Phiri, D., Simwanda, M., Salekin, S., et al., 2020. Sentinel-2 data for land cover/use mapping: A review. Remote sensing. 12(14), 2291. DOI: https://doi.org/10.3390/rs12142291
[27] Spoto, F., Sy, O., Laberinti, P., et al., 2012. Overview of sentinel-2. 2012 IEEE international geoscience and remote sensing symposium; 22-27 July 2012; Munich, Germany. pp. 1707–1710.
[28] Ferrari, F., Ferreira, M.P., Feitosa, R.Q., 2023. FUSING SENTINEL-1 AND SENTINEL-2 IMAGES WITH TRANSFORMER-BASED NETWORK FOR DEFORESTATION DETECTION IN THE BRAZILIAN AMAZON UNDER DIVERSE CLOUD CONDITIONS. ISPRS Annals of the Photogrammetry. Remote Sensing and Spatial Information Sciences. 10, 999–1006. DOI: https://doi.org/10.5194/isprs-annals-X-1-W1-2023-999-2023
[29] Darbari, P., Kumar, M., Agarwal, A., 2024. SIT.net: SAR Deforestation Classification of Amazon Forest for Land Use Land Cover Application. Journal of Computer and Communications. 12(3), 68–83.
[30] Darbari, P., Kumar, M., Agarwal, A., 2024. Satellite Image Enhancement Optical and Sar Image Fusion for Lulc Application: Comprehensive Review for State of Art. TuijinJishu/Journal of Propulsion Technology. 45(1), 1891–1906.
[31] Darbari, P., Agarwal, A., Kumar, M., 2025. SiM: Satellite Image Mixed Pixel Deforestation Analysis in Optical Satellite for Land Use Land Cover Application. Journal of Environmental & Earth Sciences. 7(2), 228–247. DOI: https://doi.org/10.30564/jees.v7i2.7737
[32] Mngadi, M., Odindi, J., Peerbhay, K., et al., 2021. Examining the effectiveness of Sentinel-1 and 2 imagery for commercial forest species mapping. Geocarto International. 36(1), 1–12.
[33] Marujo, R.F.B., Fonseca, L.M.G., Körting, T.S., et al., 2020. A multi-scale segmentation approach to filling gaps in landsat ETM+ SLC-off images through pixel weighting. International Archives of the Photogrammetry, Remote Sensing and Spatial Information Sciences. 42, 79–84.
[34] Ortega Adarme, M., Queiroz Feitosa, R., Nigri Happ, P., et al., 2020. Evaluation of deep learning techniques for deforestation detection in the Brazilian Amazon and cerrado biomes from remote sensing imagery. Remote Sensing. 12(6), 910. DOI: https://doi.org/10.3390/rs12060910
[35] Torres, D.L., Turnes, J.N., Soto Vega, P.J., et al., 2021. Deforestation Detection with Fully Convolutional Networks in the Amazon Forest from Landsat-8 and Sentinel-2 Images. Remote Sensing. 13(24), 5084. DOI: https://doi.org/10.3390/rs13245084
[36] Fonseca, L.M., Körting, T.S., Bendini, H.D.N., et al., 2021. Pattern recognition and remote sensing techniques applied to land use and land cover mapping in the Brazilian Savannah. Pattern recognition letters. 148, 54–60.
[37] De Luca, G., Silva, J.M.N., Di Fazio, S., et al., 2022. Integrated use of Sentinel-1 and Sentinel-2 data and open-source machine learning algorithms for land cover mapping in a Mediterranean region. European Journal of Remote Sensing. 55(1), 52–70.
[38] Pacheco-Pascagaza, A.M., Gou, Y., Louis, V., et al., 2022. Near real-time change detection system using Sentinel-2 and machine learning: a test for Mexican and Colombian forests. Remote Sensing. 14(3), 707. DOI: https://doi.org/10.3390/rs14030707
[39] Silva, C.A., Guerrisi, G., Del Frate, F., et al., 2022. Near-real time deforestation detection in the Brazilian Amazon with Sentinel-1 and neural networks. European Journal of Remote Sensing. 55(1), 129–149.
[40] Saha, S., Bhattacharjee, S., Shit, P.K., et al., 2022. Deforestation probability assessment using integrated machine learning algorithms of Eastern Himalayan foothills (India). Resources, Conservation & Recycling Advances. 14, 200077. DOI: https://doi.org/10.1016/j.rcradv.2022.200077
[41] Matosak, B.M., Fonseca, L.M.G., Taquary, E.C., et al., 2022. Mapping Deforestation in Cerrado Based on Hybrid Deep Learning Architecture and Medium Spatial Resolution Satellite Time Series. Remote sensing. 14(1), 209. DOI: https://doi.org/10.3390/rs14010209
[42] Mateen, S., Nuthammachot, N., Techato, K., 2024. Random forest and artificial neural network-based tsunami forests classification using data fusion of Sentinel-2 and Airbus Vision-1 satellites: A case study of Garhi Chandan, Pakistan. Open Geosciences. 16(1), 20220595. DOI: https://doi.org/10.1515/geo-2022-0595
[43] Kuzu, R.S., Antropov, O., Molinier, M., et al., 2024. Forest Disturbance Detection via Self-supervised and Transfer Learning with Sentinel-1&2 Images. IEEE Journal of Selected Topics in Applied Earth Observations and Remote Sensing. 17, 4751–4767.
[44] Gedle, A., Rientjes, T., Haile, A., 2024. Integrating Temporal-aggregated Satellite Image with Multi-Sensor Image Fusion for Seasonal Land-Cover Mapping of Shilansha Watershed, Rift Valley Basin of Ethiopia. Remote Sensing Applications: Society and Environment. 36, 101320. DOI: https://doi.org/10.1016/j.rsase.2024.101320
[45] Zhu, X., Bao, W., 2024. Performance Comparison of Image Fusion Alternatives Combining PCA with Multi-resolution Wavelet Transforms. Journal of the Indian Society of Remote Sensing. 52(5), 943–956.
[46] Li, Z., Ota, T., Mizoue, N., 2024. Monitoring tropical forest change using tree canopy cover time series obtained from Sentinel-1 and Sentinel-2 data. International Journal of Digital Earth. 17(1), 2312222. DOI: https://doi.org/10.1080/17538947.2024.2312222
[47] Garcia del Real, J., Alcaraz, M., 2024. A quick and cost-effective method for monitoring deforestation of oil sands mining activities using Synthetic Aperture Radar and Multispectral real-time satellite data from Sentinel-1 and Sentinel-2. Journal of Sustainable Mining. 23(1), 61–86.
[48] Darbari, P., Kumar, M., 2022. Satellite Image Enhancement Techniques: A Comprehensive Review. In: Goyal, V., Gupta, M., Mirjalili, S., et al. (eds.). Proceedings of International Conference on Communication and Artificial Intelligence. Lecture Notes in Networks and Systems, vol 435. Springer, Singapore. pp. 431–447. DOI: https://doi.org/10.1007/978-981-19-3571-8_36
[49] Li, J., Zhang, J., Yang, C., Liu, H., Zhao, Y., & Ye, Y. (2023). Comparative analysis of pixel-level fusion algorithms and a new high-resolution dataset for SAR and optical image fusion. Remote Sensing, 15(23), 5514.
[50] Pande, S., 2023. Land use/land cover classification of fused Sentinel-1 and Sentinel-2 imageries using ensembles of Random Forests [Thesis for Master of Technology]. Indian Institute of Technology Kanpur: Kanpur, India. DOI: https://doi.org/10.48550/arXiv.2312.10798
Downloads
How to Cite
Issue
Article Type
License
Copyright © 2025 Priyanka Darbari, Ankush Agarwal , Manoj Kumar

This is an open access article under the Creative Commons Attribution-NonCommercial 4.0 International (CC BY-NC 4.0) License.