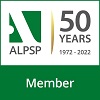
Machine Learning and Regression Analysis Reveal Different Patterns of Influence on Net Ecosystem Exchange at Two Conifer Woodland Sites
DOI:
https://doi.org/10.30564/re.v4i2.4552Abstract
Variations in net ecosystem exchange (NEE) of carbon dioxide, and the variables influencing it, at woodland sites over multiple years determine the long term performance of those sites as carbon sinks. In this study, weekly-averaged data from two AmeriFlux sites in North America of evergreen woodland, in different climatic zones and with distinct tree and understory species, are evaluated using four multi-linear regression (MLR) and seven machine learning (ML) models. The site data extend over multiple years and conform to the FLUXNET2015 pre-processing pipeline. Twenty influencing variables are considered for site CA-LP1 and sixteen for site US-Mpj. Rigorous k-fold cross validation analysis verifies that all eleven models assessed generate reproducible NEE predictions to varying degrees of accuracy. At both sites, the best performing ML models (support vector regression (SVR), extreme gradient boosting (XGB) and multi-layer perceptron (MLP)) substantially outperform the MLR models in terms of their NEE prediction performance. The ML models also generate predicted versus measured NEE distributions that approximate cross-plot trends passing through the origin, confirming that they more realistically capture the actual NEE trend. MLR and ML models assign some level of importance to all influential variables measured but their degree of influence varies between the two sites. For the best performing SVR models, at site CA-LP1, variables air temperature, shortwave radiation outgoing, net radiation, longwave radiation outgoing, shortwave radiation incoming and vapor pressure deficit have the most influence on NEE predictions. At site US-Mpj, variables vapor pressure deficit, shortwave radiation incoming, longwave radiation incoming, air temperature, photosynthetic photon flux density incoming, shortwave radiation outgoing and precipitation exert the most influence on the model solutions. Sensible heat exerts very low influence at both sites. The methodology applied successfully determines the relative importance of influential variables in determining weekly NEE trends at both conifer woodland sites studied.
Keywords:
Eddy covariance; FLUXNET2015; Weekly NEE trends; Variable importance; Correlation comparisons; NEE predictionReferences
[1] Baldocchi, D.D., Hicks, B.B., Meyers, T.P., 1988. Measuring biosphere-atmosphere exchanges of biologically related gases with micrometeorological methods. Ecology. 69, 1331-1340.
[2] Swinbank, W.C., 1951. The measurement of vertical transfer of heat and water vapor by eddies in the lower atmosphere. Journal of Meteorology. 8(3), 135- 145. DOI: https://doi.org/10.1175/1520-0469(1951)008%3C0135:TMOVTO%3E2.0.CO;2
[3] Valentini, R., 2003. Fluxes of carbon, water and energy of European forests. Ecological Studies. pp. 270. DOI: https://doi.org/10.1007/978-3-662-05171-9
[4] Goulden, M.L., Munger, W., Fan, S.M., Daube, B.C., Wofsy, S.C., 1996. Measurements of carbon sequestration by long-term eddy covariance: methods and a critical evaluation of accuracy. Global Change Biology. 2(3),169-182. DOI: https://doi.org/10.1111/j.1365-2486.1996.tb00070.x
[5] Barnhart, B.L., Eichinger, W.E., Prueger, J.H., 2012. A new eddy-covariance method using empirical mode decomposition. Boundary Layer Meteorology. 145(2), 369-382. DOI: https://doi.org/10.1007/s10546-012-9741-6
[6] Baldocchi, D.D., 2020. How eddy covariance flux measurements have contributed to our understanding of global change biology. Global Change Biology. 26, 242-260.
[7] Baldocchi, D., Chu, H., Reichstein, M., 2018. Inter-annual variability of net and gross ecosystem carbon fluxes: a review. Agriculture and Forest Meteorology. 249, 520-533. DOI: https://doi.org/10.1016/j.agrformet.2017.05.015
[8] Monteith, J.L., 1972. Solar radiation and productivity in tropical ecosystems. Journal of Applied Ecology. 9(3), 747. DOI: https://doi.org/10.2307/2401901
[9] Saigusa, N., Yamamoto, S., Murayama, S., Kondo, H., Nishimura, N., 2002. Gross primary production and net ecosystem exchange of a cool-temperate deciduous forest estimated by the eddy covariance method. Agricultural and Forest Meteorology. 112(3-4), 203- 215. DOI: https://doi.org/10.1016/S0168-1923(02)00082-5
[10] Sellers, P.J., Berry, J.A., Collatz, G.J., Field, C.B., Hall., F.G., 1992. Canopy reflectance, photosynthesis, and transpiration. III. a reanalysis using improved leaf models and a new canopy integration scheme. Remote Sensing of Environment. 42(3), 187-216. DOI: https://doi.org/10.1016/0034-4257(92)90102-P
[11] Chu, H., Baldocchi, D.D., Poindexter, C., Abraha, M., Desai, A.R., Bohrer, G., Arain, M.A., et al., 2018. Temporal dynamics of aerodynamic canopy height derived from eddy covariance momentum flux data across North American flux networks Geophysical Research Letters. 45, 9275-9287. DOI: https://doi.org/10.1029/2018GL079306
[12] Holtmann, A., Huth, A., Pohl, F., Rebmann, C., Fischer, R., 2021. Carbon Sequestration in Mixed Deciduous Forests: The Influence of Tree Size and Species Composition Derived from Model Experiments. Forests. 12, 726. DOI: https://doi.org/10.3390/f12060726
[13] Falge, E., Aubinet, M., Bakwin, P., Baldocchi, D., Berbigier, P., Bernhofer, C., Black, T., et al., 2005. FLUXNET Marconi conference gap-filled flux and meteorology data, 1992-2000. https://catalog.data.gov/dataset/fluxnet-marconi-conference-gap-filled-flux-and-meteorology-data-1992-2000 (Accessed 20th March 2022)
[14] Neog, P., Kumar, A., Srivastava, A.K., Chakravarty, N.V.K., 2005. Estimation and application of Bowen ratio fluxes over crop surfaces - an overview. Journal of Agricultural Physics. 5(1), 36-45.
[15] Yuan, W., Liu, S., Zhou, G., Zhou, G., Tieszen, L.L., Baldocchi, D., Bernhofer, C., et al., 2007. Deriving a light use efficiency model from eddy covariance flux data for predicting daily gross primary production across biomes. Agricultural and Forest Meteorology. 143(3-4), 189-207. DOI: https://doi.org/10.1016/J.AGRFORMET.2006.12.001
[16] Ge, S., Smith, R.G., Jacovides, C.P., Kramer, M.G., Carruthers, R.I., 2011. Dynamics of photosynthetic photon flux density (PPFD) and estimates in coastal northern California. Theoretical and Applied Climatology. 105, 107-118. DOI: https://doi.org/10.1007/s00704-010-0368-6
[17] Kia, S.H., Milton, E.J., 2015. Hyper-temporal remote sensing for scaling between spectral indices and flux tower measurements. Applied Ecology and Environmental Research. 13(2), 465-487. DOI: https://doi.org/10.15666/aeer/1302_465487
[18] Tucker, C.J., 1979. Red and photographic infrared linear combinations for monitoring vegetation. Remote Sensing of Environment. 8(2), 127-150.
[19] Niu, B., He, Y., Zhang, X., Fu, G., Shi, P., Du, M., Zhang, Y., Zong, N., 2016. Tower-based validation and improvement of MODIS gross primary production in an alpine swamp meadow on the Tibetan Plateau. Remote Sensing. 8(7), 592. DOI: https://doi.org/10.3390/rs8070592
[20] Xu, C., Qu, J.J., Hao, X., Zhu, Z., Gutenberg, L., 2020. Monitoring soil carbon flux with in-situ measurements and satellite observations in a forested region. Geoderma. 378, 114617. DOI: https://doi.org/10.1016/j.geoderma.2020.114617
[21] Zhou, X., Wang, X., Tong, L., Zhang, H., Lu, F., Zheng, F., Hou, P., Song, W., Ouyang, Z., 2012. Soil warming effect on net ecosystem exchange of carbon dioxide during the transition from winter carbon source to spring carbon sink in a temperate urban lawn. Journal of Environmental Sciences (China). 24(12), 2104-2112. DOI: http://www.ncbi.nlm.nih.gov/pubmed/23534206
[22] Valentini, R., Matteucci, G., Dolman, A.J., Schulze, E.D., Rebmann, C., Moors, E.J., Granier, A., et al., 2000. Respiration as the main determinant of carbon balance in European forests. Nature. 404(6780), 861- 865. DOI: https://doi.org/10.1038/35009084
[23] Zhu, S., Clement, R., McCalmont, J., Davies, C.A., Hill, T., 2022. Stable gap-filling for longer eddy covariance data gaps: A globally validated machine-learning approach for carbon dioxide, water, and energy fluxes. Agricultural and Forest Meteorology. 314(1), 108777. DOI: https://doi.org/10.1016/j.agrformet.2021.108777
[24] Rödig, E., Huth, A., Bohn, F., Rebmann, C., Cuntz, M., 2017. Estimating the carbon fluxes of forests with an individual-based forest model. Forest Ecosystems. 4, 4. DOI: https://doi.org/10.1186/s40663-017-0091-1
[25] Duman, T., Schäfer, K.V.R., 2018. Partitioning net ecosystem carbon exchange of native and invasive plant communities by vegetation cover in an urban tidal wetland in the New Jersey Meadowlands (USA). Ecological Engineering. 114, 16-24. DOI: https://doi.org/10.1016/J.ECOLENG.2017.08.031
[26] Churkina, G., Schimel, D., Braswell, B.H., Xiao, X., 2005. Spatial analysis of growing season length control over net ecosystem exchange. Global Change Biology. 11(10), 1777-1787. DOI: https://doi.org/10.1111/j.1365-2486.2005.001012.x
[27] Mendes, K.R., Suany Campos, S., da Silva, L.L., Mutti, P.R., Ferreira, R.R., Medeiros, S.S., et al., 2020. Seasonal variation in net ecosystem CO2 exchange of a Brazilian seasonally dry tropical forest. Scientific Reports. 10, 9454. DOI: https://doi.org/10.1038/s41598-020-66415-w
[28] Wood, D.A., 2022. Net Ecosystem Exchange Comparative Analysis of the Relative Influence of Recorded Variables in Well Monitored Ecosystems. Ecological Complexity. 50, 100998. DOI: https://doi.org/10.1016/j.ecocom.2022.100998
[29] Cai, J., Xu, K., Zhu, Y., Hu, F., Li, L., 2020. Prediction and analysis of net ecosystem carbon exchange based on gradient boosting regression and random forest. Applied Energy. 262, 114566. DOI: https://doi.org/10.1016/j.apenergy.2020.114566
[30] Abbasian, H., Solgia, E., Hosseini, S.M., Kia, H., 2022. Modeling terrestrial net ecosystem exchange using machine learning techniques based on flux tower measurements. Ecological Modelling. 446, 109901. DOI: https://doi.org/10.1016/j.ecolmodel.2022.109901
[31] Wood, D.A., 2021. Net ecosystem carbon exchange prediction and data mining with an optimized data-matching algorithm achieves useful knowledge-based learning relevant to environmental carbon storage. Ecological Indicators. 124, 107426. DOI: https://doi.org/10.1016/j.ecolind.2021.107426
[32] AmeriFlux, 2022. AmeriFlux Management Project. https://ameriflux.lbl.gov/about/ameriflux-management-project/ (Accessed 20th March 2022)
[33] FLUXNET, 2022. International network of eddy covariance measurement sites. https://fluxnet.org/ (Accessed 20th March 2022)
[34] Kirschbaum, M.U., Mueller, R., 2001. Net ecosystem exchange: workshop proceedings, cooperative research centre for greenhouse accounting. pp. 136. https://www.kirschbaum.id.au/NEE_Workshop_Proceedings.pdf (Accessed 20th March 2022)
[35] Reichstein, M., Falge, E.M., Baldocchi, D.D., Papale, D., Aubinet, M., Berbigier, P., et al., 2005. On the separation of net ecosystem exchange into assimilation and ecosystem respiration: review and improved algorithm. Global Change Biology. 11, 1424-1439. DOI: https://doi.org/10.1111/j.1365-2486.2005.001002.x
[36] Luyssaert, S., Reichstein, M., Schulze, E-D., Janssens, A., Law, B.E., Papale, D., et al., 2009. Toward a consistency cross-check of eddy covariance fluxbased and biometric estimates of ecosystem carbon balance. Global Biogeochemical Cycles. 23, 13. DOI: https://doi.org/10.1029/2008GB003377
[37] Fei, X., Jin, Y., Zhang, Y., Sha, L., Liu, Y., Song, Q., Zhou, W., Liang, N., Yu, G., Zhang, L., Zhou, R., Li, J., Zhang, S., Li, P., 2017. Eddy covariance and biometric measurements show that a savanna ecosystem in Southwest China is a carbon sink. Scientific Reports. 7, 41025. DOI: https://doi.org/10.1038/srep41025
[38] Baldocchi, D., Falge, E., Gu, L., et al., 2001. FLUXNET: A new tool to study the temporal and spatial variability of ecosystem-scale carbon dioxide, water vapor, and energy flux densities. Bulletin of the American Meteorological Society. 82(82), 2415-2434.
[39] Pastorello, G., Trotta, C., Canfora, E., et al., 2020. The FLUXNET2015 dataset and the ONEFlux processing pipeline for eddy covariance data. Scientific Data. 7, 225. DOI: https://doi.org/10.1038/s41597-020-0534-3
[40] Ameriflux, 2022. Flux/met data processing pipeline overview. https://ameriflux.lbl.gov/data/data-processing-pipelines/ (Accessed 20th March 2022).
[41] Ameriflux, 2022. Data variable descriptions for the FLUXNET product. https://ameriflux.lbl.gov/data/aboutdata/data-variables/ (Accessed 20th March 2022).
[42] Black, T.A., 2021. AmeriFlux FLUXNET-1F CALP1 British Columbia - Mountain pine beetle-attacked lodgepole pine stand. AmeriFlux AMP, (Dataset). DOI: https://doi.org/10.17190/AMF/1832155
[43] Brown, M., Black, T.A., Nesic, Z., Foord, V.N., Spittlehouse, D.L., Fredeen, A.L., Grant, N.J., Burton, P.J., Trofymow, J.A., 2010. Impact of mountain pine beetle on the net ecosystem production of lodgepole pine stands in British Columbia. Agricultural & Forest Meteorology. 150(2), 254-264. DOI: https://doi.org/10.1016/j.agrformet.2009.11.008
[44] Litvak, M., 2021. AmeriFlux FLUXNET-1F US-Mpj Mountainair Pinyon-Juniper Woodland. AmeriFlux AMP, (Dataset). DOI: https://doi.org/10.17190/AMF/1832161
[45] Morillas, L., Pangle, R.E., Maurer, G.E., Pockman, W.T., McDowell, N., Huang, C., Krofcheck, D.J., Fox, A.M., Sinsabaugh, R.L., Rahn, T.A., Litvak, M.E., 2017. Tree mortality decreases water availability and ecosystem resilience to drought in piñon-juniper woodlands in the southwestern U.S. Journal of Geophysical Research: Biogeosciences. 122(12), 3343-3361. DOI: https://doi.org/10.1002/2017JG004095
[46] Pearson, K., 1894. On the dissection of asymmetrical frequency curves. Philosophical Transactionsof the Royal Society of London. 185, 71-110.
[47] Spearman, C., 1904. The proof and measurement of association between two things. American Journal of Psychology. 15(1), 72-101. DOI: https://doi.org/10.2307/1412159
[48] Lawrence, I., Lin, K., 1989. A concordance correlation coefficient to evaluate reproducibility. Biometrics. pp. 255-268. DOI: https://doi.org/10.2307/2532051
[49] Boddy, R., Smith, G., 2009. Statistical Methods in Practice: For scientists and technologists. Chichester, U.K.: Wiley. pp. 95-96.
[50] Wayne, D.W., 1990. Spearman rank correlation coefficient. Applied Nonparametric Statistics (2nd ed.). Boston: PWS-Kent.
[51] Myers, L., Sirois, M.J., 2004. Spearman correlation coefficients, differences between. Encyclopedia of Statistical Sciences. DOI: https://doi.org/10.1002/0471667196.ess5050
[52] Artusi, R., Verderio, P., Marubini, E., 2002. Bravais-Pearson and Spearman correlation coefficients: meaning, test of hypothesis and confidence interval. The International Journal of Biological Markers. 17(2), 148-151. DOI: https://journals.sagepub.com/doi/pdf/10.1177/172460080201700213
[53] Harrell, F.E., 2015. Regression Modeling Strategies. Second Edition. Springer, Switzerland. pp. 582. DOI: https://doi.org/10.1007/978-3-319-19425-7
[54] Goldberger, A.S., 1964. Classical linear regression. Econometric Theory. New York: John Wiley & Sons. pp. 158.
[55] Stigler, S.M., 1981. Gauss and the Invention of Least Squares. Annals of Statistics. 9(3), 465-474. DOI: https://doi.org/10.1214/aos/1176345451
[56] Bottou, L., 1998. Online algorithms and stochastic approximations. Online Learning and Neural Networks. Cambridge University Press.
[57] SciKit Learn, 2022. Linear models. https://scikitlearn.org/stable/modules/linear_model.html (Accessed 20th March 2022).
[58] SciKit Learn, 2022. Supervised and unsupervised machine learning models in Python. 2022a. https://scikit-learn.org/stable/ (Accessed 20th March 2022).
[59] Freund, Y., Schapire, R.E., 1997. A decision-theoretic generalization of on-line learning and an application to boosting. Journal of Computer and System Sciences. 55, 119-139. DOI: https://doi.org/10.1006/jcss.1997.1504
[60] Chan, J.C.W., Paelinckx, D., 2008. Evaluation of Random Forest and Adaboost tree-based ensemble classification and spectral band selection for ecotope mapping using airborne hyperspectral imagery. Remote Sensing of Environment. 112(6), 2999-3011. DOI: https://doi.org/10.1016/j.rse.2008.02.011
[61] Quinlan, J.R., 1986. Induction of decision trees. Machine Learning. 1, 81-106. DOI: https://doi.org/10.1007/BF00116251
[62] Debeljak, M., Džeroski, S., 2011. Decision trees in ecological modelling. Modelling complex ecological dynamics. Springer, Berlin, Heidelberg. pp. 197-209.
[63] Fix, E., Hodges Jr., J.L., 1951. Discriminatory analysis, nonparametric discrimination: consistency properties. Technical Report, USAF School of Aviation Medicine.
[64] Fu, Y., He, H.S., Hawbaker, T.J., Henne, P.D., Zhu, Z., Larsen, D.R., 2019. Evaluating k-Nearest Neighbor (kNN) imputation models for species-level aboveground forest biomass mapping in northeast China. Remote Sensing. 11, 2005. DOI: https://doi.org/10.3390/rs11172005
[65] Rosenblatt, F., 1958. The Perceptron: A Probabilistic Model for Information Storage and Organization in the Brain, Cornell Aeronautical Laboratory. Psychological Review. 65(6), 386-408. DOI: https://doi.org/10.1037/h0042519
[66] Eshel, G., Dayalu, A., Wofsy, S.C.C., Munger, J.W., Tziperman, E., 2019. Listening to the forest: An artificial neural network-based model of carbon uptake at Harvard Forest, Journal of Geophysical Research: Biogeosciences. 124, 461-478. DOI: https://doi.org/10.1029/2018JG004791
[67] Safa, B., Arkebauer, T.J., Zhu, Q., Suyker, A., Irmak, S., 2019. Net Ecosystem Exchange (NEE) simulation in maize using artificial neural networks. IFAC Journal of Systems and Control. 7, 100036. DOI: https://doi.org/10.1016/j.ifacsc.2019.100036
[68] Ho, T.K., 1998. The random subspace method for constructing decision forests. IEEE Transactions on Pattern Analysis and Machine Intelligence. 20(8), 832-844. DOI: https://doi.org/10.1109/34.709601
[69] Zhou, O., Fellows, A., Flerchinger, G.N., Flores, A.N., 2019. Examining interactions between and among predictors of net ecosystem exchange: a machine learning approach in a semi-arid landscape. Scientific Reports. 9, 2222. DOI: https://doi.org/10.1038/s41598-019-38639-y
[70] Huang, N., Wang, L., Zhang, Y., Gao, S., Niu, Z., 2021. Estimating the net ecosystem exchange at global FLUXNET sites using a random forest model. In IEEE Journal of Selected Topics in Applied Earth Observations and Remote Sensing. 14, 9826-9836. DOI: https://doi.org/10.1109/JSTARS.2021.3114190
[71] Cortes, C., Vapnik, V., 1995. Support-Vector Networks. Machine Learning. 20(3), 273-297. DOI: https://doi.org/10.1007/BF00994018
[72] IIlie, I., Dittrich, P., Carvalhais. N., Jung, M., Heinemeyer, A., Migliavacca, M., Morison, J.I.L., Sippel, S., Subke, J.A., Wilkinson, M., Mahecha, M.D., 2017. Reverse engineering model structures for soil and ecosystem respiration: the potential of gene expression programming. Geoscientific Model Development. 10(9), 3519-3545. DOI: https://doi.org/10.5194/gmd-10-3519-2017
[73] Li, Z., Chen, C., Nevins, A., Pirtle, T., Cui, S., 2021. Assessing and modeling ecosystem carbon exchange and water vapor flux of a pasture ecosystem in the temperate climate-transition zone. Agronomy. 11, 2071. DOI: https://doi.org/10.3390/agronomy11102071
[74] Chen, T., Guestrin, C., 2016. XGBoost: a scalable tree boosting system. In Krishnapuram, Balaji; Shah, Mohak; Smola, Alexander J.; Aggarwal, Charu C.; Shen, Dou; Rastogi, Rajeev (eds.). Proceedings of the 22nd ACM SIGKDD International Conference on Knowledge Discovery and Data Mining, San Francisco, CA, USA. ACM. pp. 785-794. DOI: https://doi.org/10.1145/2939672.2939785
[75] Yan, S., Wu, L., Zhang, F., Zou, Y., Wu, Y., 2021. A novel hybrid WOA-XGB model for estimating daily reference evapotranspiration using local and external meteorological data: Applications in arid and humid regions of China. Agricultural Water Management. 244, 106594. DOI: https://doi.org/10.1016/j.agwat.2020.106594
[76] Liu, J., Zuo, Y., Wang, N., Yuan, F., Zhu, X., Zhang, L., Zhang, J., Sun, Y., Guo, Z., Guo, Y., Song, X., Song, C., Xu, X.F., 2021. Comparative analysis of two machine learning algorithms in predicting site-level net ecosystem exchange in major biomes. Remote Sensing. 13, 2242. DOI: https://doi.org/10.3390/rs13122242
[77] SciKit Learn. 2022 GridSearchCV: Exhaustive search over specified parameter values for an estimator in Python. https://scikit-learn.org/stable/modules/generated/sklearn.model_selection.GridSearchCV.html (Accessed 20th March 2022).
[78] SciKit Learn. 2022 Bayesian optimization of hyperparameters in Python.. https://scikit-optimize.github.io/stable/modules/generated/skopt.BayesSearchCV.html (Accessed 20th March 2022).
[79] SciKit Learn. 2022 Cross-validation: evaluating estimator performance. https://scikit-learn.org/stable/modules/cross_validation.html (Accessed 20th March 2022).
[80] Gini, C., 1997. Concentration and dependency ratios (published 1909 in Italian). English translation in Rivista di Politica Economica. 87, 769-778.
[81] Guillermina, J., 1979. On Gini’s Mean Difference and Gini’s Index of Concentration. American Sociological Review. 44(5), 867-870. DOI: https://doi.org/10.2307/2094535
[82] Verduzco, V.S., Garatuza-Payán, J., Yépez, E.A., Watts, C.J., Rodríguez, J.C., Robles-Morua, A., Vivoni, E.R., 2015. Variations of net ecosystem production due to seasonal precipitation differences in a tropical dry forest of northwest Mexico. Journal of Geophysical Research: Biogeosciences. 120(10), 2081-2094. DOI: https://doi.org/10.1002/2015JG003119
[83] Yepez, E.A., Garatuza, J., 2021. AmeriFlux FLUXNET-1F MX-Tes Tesopaco, secondary tropical dry forest, Ver. 3-5, AmeriFlux AMP, (Dataset). DOI: https://doi.org/10.17190/AMF/1832156
[84] Griffis, T., Roman, D., Wood, J., Deventer, J., Fachin, L., Rengifo, J., Del Castillo, D., Lilleskov, E., Kolka, R., Chimner, R., del Aguila-Pasquel, J., Wayson, C., Hergoualc’h, K., Baker, J., Cadillo-Quiroz, H., Ricciuto, D., 2020. Hydrometeorological sensitivities of net ecosystem carbon dioxide and methane exchange of an Amazonian palm swamp peatland agricultural and forest meteorology. 295, 108167. DOI: https://doi.org/10.1016/j.agrformet.2020.108167
[85] Schulze, E.D., Valentini, R., Bouriaud, O., 2021. The role of net ecosystem productivity and of inventories in climate change research: the need for net ecosystem productivity with harvest (NEPH). Forest Ecosystems. 8, 15. DOI: https://doi.org/10.1186/s40663-021-00294-z
[86] Cable, J., Ogle, K., Barron-Gafford, G., Bentley, L., Cable, W., Scott, R., Williams, D., Huxman, T., 2013. Antecedent conditions influence soil respiration differences in shrub and grass patches. Ecosystems. 16, 1230-1247.
[87] Wiesner, S., Staudhammer, C.L., Javaheri, C.L., Kevin Hiers, J.K., Boring, L.R., Mitchell, R.J., Starr, G., 2019. The role of understory phenology and productivity in the carbon dynamics of longleaf pine savannas. Ecosphere. 10(4), e02675. DOI: https://doi.org/10.1002/ecs2.2675
[88] Matusick, G., Hudson, S.J., Garrett, C.Z., Samuelson, L.J., Kent, J.D., Addington, R.N., Parker, J.M., 2020. Frequently burned loblolly-shortleaf pine forest in the southeastern United States lacks the stability of longleaf pine forest. Ecosphere. 11(2), e03055. DOI: https://doi.org/10.1002/ecs2.3055
Downloads
How to Cite
Issue
Article Type
License
Copyright © 2022 The author(s)

This is an open access article under the Creative Commons Attribution-NonCommercial 4.0 International (CC BY-NC 4.0) License.