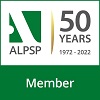
Determining Learning Style Preferences of Learners
DOI:
https://doi.org/10.30564/jcsr.v3i1.2761Abstract
The use of Information and Communication Technology (ICT) in education has been rapidly growing in recent years which has converted conventional classrooms teaching environments into online learning (OL) environment. Online learning system is gaining popular and widely accepted in the world due to the current pandemic due to COVID 19. This has created an opportunity to take online classes through several online learning platforms. This research was also done during pandemic. The data were collected from one of the undergraduate courses where there were 108 learners. The objective of the study is to determine the learning style preferences based on the learner’s interactions data. one of the popular and widely used learning style model called Felder Silverman Learning Style Model (FSLSM) was implemented in this study to determine the learning preferences. The learners were classified according to the two dimensions i.e., input and processing of FSLSM. Further, two popular treebased classifier such as decision tree and random forest were implemented. Decision tree had a better performance in terms of accuracy than random forest. This type of research is very much beneficial to the instructors, learners and researchers and administrators working in the field of online learning.
This article has been updated.
A correction to this article was published on January 25, 2022 and can be found at https://doi.org/10.30564/jcsr.v4i1.4400
Keywords:
Online learning; Moodle; FSLSM; Data mining; Decision tree; Random forestReferences
[1] Deborah, L. J., Baskaran, R., Kannan, A. Learning styles assessment and theoretical origin in an E-learning scenario: a survey. Artificial Intelligence Review, 2014, 42(4): 801-819. https://doi.org/10.1007/s10462-012-9344-0
[2] Shute, V., Towle, B. Adaptive e-learning. Educational psychologist, 2003, 38(2): 105-114. https://doi.org/10.1207/S15326985EP3802_5
[3] Pashler, H., McDaniel, M., Rohrer, D., Bjork, R. Learning styles: concepts and evidence. Psychological Science in the Public Interest, 2009, 9(3): 105- 119. https://doi.org/10.1111%2Fj.1539-6053.2009.01038.x
[4] Graf, S. Enabling learning management systems to identify learning styles, 2006. CiteSeerX — Enabling Learning Management Systems to Identify Learning Styles * (psu.edu)
[5] Graf, S., Viola, S. R., Leo, T., Kinshuk. In-depth analysis of the Felder-Silverman learning style dimensions. Journal of Research on Technology in Education, 2007, 40(1): 79-93. https://doi.org/10.1080/15391523.2007.10782498
[6] Felder, R. M. Felder-Soloman Index of Learning Styles[Online] ,2013. Available: http://www4.ncsu.edu/unity/lockers/users/f/felder/public.ILSpage.html
[7] Felder, R. M., Silverman, L. K. Learning and teaching styles in engineering education. Engineering Education, 1988, 78(7): 674-681. [PDF] Learning and Teaching Styles in Engineering Education. | Semantic Scholar
[8] Liyanage, M. P. P., KS, L. G., Hirakawa, M. Detecting learning styles in learning management systems using data mining. Journal of Information Processing, 2017, 24(4): 740-749. https://doi.org/10.2197/ipsjjip.24.740
[9] Maaliw III, R. R., Ballera, M. A. Classification of Learning Styles in Virtual Learning Environment Using J48 Decision Tree. International Association for Development of the Information Society, 2017. ERIC - ED579490 - Classification of Learning Styles in Virtual Learning Environment Using J48 Decision Tree, International Association for Development of the Information Society, 2017-Oct
[10] Sheeba, T., Krishnan, R. Automatic detection of students learning style in Learning Management System. In Smart Technologies and Innovation for a Sustainable Future. Springer, Cham, 2019: 45-53. Automatic Detection of Students Learning Style in Learning Management System | SpringerLink
[11] Romero, C., Ventura, S., García, E. Data mining in course management systems: Moodle case study and tutorial. Computers & Education, 2008, 51(1): 368- 384. https://doi.org/10.1016/j.compedu.2007.05.016
[12] El Aissaoui, O., El Alami, Y. E. M., Oughdir, L., El Allioui, Y. A hybrid machine learning approach to predict learning styles in adaptive E-learning system. In International Conference on Advanced Intelligent Systems for Sustainable Development. Springer, Cham, 2018: 772-786. A Hybrid Machine Learning Approach to Predict Learning Styles in Adaptive E-Learning System | SpringerLink
[13] Bernard, J., Chang, T. W., Popescu, E., Graf, S. Learning style Identifier: Improving the precision of learning style identification through computational intelligence algorithms. Expert Systems with Applications, 2017, 75: 94-108. https://doi.org/10.1016/j.eswa.2017.01.021
[14] Kolekar, S. V., Pai, R. M., MM, M. P. Rule based adaptive user interface for adaptive E-learning system. Education and Information Technologies, 2019, 24(1): 613-641. Rule based adaptive user interface for adaptive E-learning system | SpringerLink
[15] El Fazazi, H., Samadi, A., Qbadou, M., Mansouri, K., Elgarej, M. A Learning Style Identification Approach in Adaptive E-Learning System. In International Conference Europe Middle East & North Africa Information Systems and Technologies to Support Learning. Springer, Cham, 2018: 82-89. A Learning Style Identification Approach in Adaptive E-Learning System | SpringerLink
[16] El Aissaoui, O., El Madani, Y. E. A., Oughdir, L., El Allioui, Y. Combining supervised and unsupervised machine learning algorithms to predict the learners’ learning styles. Procedia computer science, 2019, 148: 87-96. A Learning Style Identification Approach in Adaptive E-Learning System | SpringerLink
[17] Hew, K. F., Hu, X., Qiao, C., Tang, Y. What predicts student satisfaction with MOOCs: a gradient boosting trees supervised machine learning and sentiment analysis approach. Computers & Education, 2019, 145: 103724. https://doi.org/10.1016/j.compedu.2019.103724
[18] Lee, M. J., Ferwerda, B. Personalizing online educational tools. In Proceedings of the 2017 ACM Workshop on Theory-Informed User Modeling for Tailoring and Personalizing Interfaces, 2017: 27-30.
[19] Yu, H., Miao, C., Leung, C., White, T. J. Towards AI-powered personalization in MOOC learning. npj Science of Learning, 2017, 2(1): 1-5. Towards AI-powered personalization in MOOC learning | npj Science of Learning (nature.com)
[20] El Ghouch, N., El Mokhtar, E.-N., Seghroucheni, Y. Z. Analysing the outcome of a learning process conducted within the system ALS_CORR [LP]. International Journal of Emerging Technologies in Learning, 2017, 12(3): 43-56. Analysing the Outcome of a Learning Process Conducted Within the System ALS_CORR[LP] | Nihad | International Journal of Emerging Technologies in Learning (iJET) (publicknowledgeproject.org)
[21] Xiao, J., Wang, M., Jiang, B., Li, J. A personalized recommendation system with combinational algorithm for online learning. Journal of Ambient Intelligence and Humanized Computing, 2018, 9(3): 667- 677. A personalized recommendation system with combinational algorithm for online learning | SpringerLink
[22] El Aissaoui, O., El Alami, Y. E. M., Oughdir, L., El Allioui, Y. Integrating web usage mining for an automatic learner profile detection: A learning stylesbased approach. In 2018 international conference on intelligent systems and computer vision (ISCV). IEEE, 2018: 1-6. https://doi.org/10.1109/ISACV.2018.8354021
[23] Azzi, I., Jeghal, A., Radouane, A., Yahyaouy, A., Tairi, H. A robust classification to predict learning styles in adaptive e-learning systems. Education and Information Technologies, 2020, 25(1): 437-448. A robust classification to predict learning styles in adaptive E-learning systems | SpringerLink
Downloads
How to Cite
Issue
Article Type
License
Copyright © 2021 Author(s)

This is an open access article under the Creative Commons Attribution-NonCommercial 4.0 International (CC BY-NC 4.0) License.