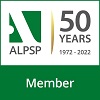
Intrusion Detection through DCSYS Propagation Compared to Auto-encoders
DOI:
https://doi.org/10.30564/jcsr.v3i3.3578Abstract
In network settings, one of the major disadvantages that threaten the network protocols is the insecurity. In most cases, unscrupulous people or bad actors can access information through unsecured connections by planting software or what we call malicious software otherwise anomalies. The presence of anomalies is also one of the disadvantages, internet users are constantly plagued by virus on their system and get activated when a harmless link is clicked on, this a case of true benign detected as false. Deep learning is very adept at dealing with such cases, but sometimes it has its own faults when dealing benign cases. Here we tend to adopt a dynamic control system (DCSYS) that addresses data packets based on benign scenario to truly report on false benign and exclude anomalies. Its performance is compared with artificial neural network auto-encoders to define its predictive power. Results show that though physical systems can adapt securely, it can be used for network data packets to identify true benign cases.
Keywords:
Dynamic control system; Deep learning; Artificial neural network; Auto-encoders; Identify space model; Benign; AnomaliesReferences
[1] Phan, N., Wang, Y., Wu, X., & Dou, D. (2016, February). Differential privacy preservation for deep auto-encoders: an application of human behavior prediction. In Thirtieth AAAI Conference on Artificial Intelligence.
[2] Kipf, T. N., & Welling, M. (2016). Variational graph auto-encoders. arXiv preprint arXiv:1611.07308.
[3] Soui, M., Smiti, S., Mkaouer, M. W., & Ejbali, R. (2020). Bankruptcy prediction using stacked auto-encoders. Applied Artificial Intelligence, 34(1), 80-100.
[4] Ding, Y., Tian, L. P., Lei, X., Liao, B., & Wu, F. X. (2021). Variational graph auto-encoders for miRNA-disease association prediction. Methods, 192, 25- 34.
[5] Wang, W., & Gómez-Bombarelli, R. (2019). Coarse-graining auto-encoders for molecular dynamics. npj Computational Materials, 5(1), 1-9.
[6] Wu, X., & Cheng, Q. (2021). Deepened Graph Auto-Encoders Help Stabilize and Enhance Link Prediction. arXiv preprint arXiv:2103.11414.
[7] Wu, X., & Cheng, Q. (2021). Deepened Graph Auto-Encoders Help Stabilize and Enhance Link Prediction. arXiv preprint arXiv:2103.11414.
[8] Gomede, E., de Barros, R. M., & de Souza Mendes, L. (2021). Deep auto encoders to adaptive e-learning recommender system. Computers and Education: Artificial Intelligence, 2, 100009.
[9] Silva, A. B. O. V., & Spinosa, E. J. (2021). Graph Convolutional Auto-Encoders for predicting novel lncRNA-Disease associations. IEEE/ACM Transactions on Computational Biology and Bioinformatics.
[10] Arifoglu, D., Wang, Y., & Bouchachia, A. (2021). Detection of Dementia-Related Abnormal Behaviour Using Recursive Auto-Encoders. Sensors, 21(1), 260.
[11] Zino, L., & Cao, M. (2021). Analysis, prediction, and control of epidemics: A survey from scalar to dynamic network models. arXiv preprint arXiv:2103.00181.
[12] Zhou, P., Chen, W., Yi, C., Jiang, Z., Yang, T., & Chai, T. (2021). Fast just-in-time-learning recursive multi-output LSSVR for quality prediction and control of multivariable dynamic systems. Engineering Applications of Artificial Intelligence, 100, 104168.
[13] Sun, C., Chen, J., Cao, S., Gao, X., Xia, G., Qi, C., & Wu, X. (2021). A Dynamic Control Strategy of District Heating Substations Based on Online Prediction and Indoor Temperature Feedback. Energy, 121228.