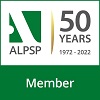
Animal Exercise: A New Evaluation Method
DOI:
https://doi.org/10.30564/jcsr.v4i2.4759Abstract
At present, Animal Exercise courses rely too much on teachers' subjective ideas in teaching methods and test scores, and there is no set of standards as a benchmark for reference. As a result, students guided by different teachers have an uneven understanding of the Animal Exercise and cannot achieve the expected effect of the course. In this regard, the authors propose a scoring system based on action similarity, which enables teachers to guide students more objectively. The authors created QMonkey, a data set based on the body keys of monkeys in the coco dataset format, which contains 1,428 consecutive images from eight videos. The authors use QMonkey to train a model that recognizes monkey body movements. And the authors propose a new non-standing posture normalization method for motion transfer between monkeys and humans. Finally, the authors utilize motion transfer and structural similarity contrast algorithms to provide a reliable evaluation method for animal exercise courses, eliminating the subjective influence of teachers on scoring and providing experience in the combination of artificial intelligence and drama education.
Keywords:
Motion transfer; Animal exercise; Evaluation method; Monkeys; Target scale normalizationReferences
[1] Stanislavski, C., 1989. An actor prepares, Routledge.
[2] Adler, S., 2000. The Art of Acting, ed. Howard Kissel (New York: Applause, 2000).
[3] Qi, Y., Zhang, C., Kameda, H., 2021. Historical summary and future development analysis of animal exercise. ICERI2021 Proceedings, 14th annual International Conference of Education, Research and Innovation. pp. 8529-8538, IATED.
[4] Aberman, K., Wu, R., Lischinski, D., et al., 2019. Learning character-agnostic motion for motion retargeting in 2d. arXiv preprint arXiv:1905.01680.
[5] Lin, T.Y., Maire, M., Belongie, S., et al., 2014. Microsoft coco: Common objects in context. European conference on computer vision. pp.740-755.
[6] Chan, C., Ginosar, S., Zhou, T., et al., 2019. Everybody dance now. Proceedings of the IEEE/CVF International Conference on Computer Vision. pp. 5933-5942.
[7] Cao, Z., Simon, T., Wei, S.E., et al., 2017. Realtimemulti-person2d pose estimation using part affinity fields. Proceedings of the IEEE conference on computer vision and pattern recognition. pp. 7291-7299.
[8] Wang, T.C., Liu, M.Y., Zhu, J.Y., et al., 2018. High-resolution image synthesis and semantic manipulation with conditional gans. Proceedings of the IEEE conference on computer vision and pattern recognition. pp. 8798-8807.
[9] Andriluka, M., Pishchulin, L., Gehler, P., et al., 2014. 2d human pose estimation: New benchmark and state of the art analysis. Proceedings of the IEEE Conference on computer Vision and PatternRecognition. pp. 3686-3693.
[10] Goodfellow, I., Pouget-Abadie, J., Mirza, M., et al., 2014. Generative adversarial nets. Advances in neural information processing systems. 27.
[11] Yoo, D., Kim, N., Park, S., et al., 2016. Pixel-level domain transfer. European conference on computer vision, Springer. pp. 517-532.
[12] Ledig, C., Theis, L., Huszár, F., et al., 2017. Photorealistic single image super-resolution using a generative adversarial network. Proceedings of the IEEE conference on computer vision and pattern recognition. pp. 4681-4690.
[13] Mirzaand, M., Osindero, S., 2014. Conditional generative adversarial nets,” arXiv preprint arXiv:1411.1784.
[14] Isola, P., Zhu, J.Y., Zhou, T., et al., 2017. Image-to-image translation with conditional adversarial networks. Proceedings of the IEEE conference on computer vision and pattern recognition. pp. 1125- 1134.
[15] Zhang, R., Isola, P., Efros, A.A., et al., 2018. The unreasonable effectiveness of deep features as a perceptual metric. Proceedings of the IEEE conference on computer vision and pattern recognition. pp. 586- 595.
[16] Wang, Z., Bovik, A.C., Sheikh, H.R., et al., 2004. Image quality assessment: from error visibility to structural similarity. IEEE transactions on image processing. 13(4), 600-612.
[17] Hore, A., Ziou, D., 2010. Image quality metrics: Psnr vs. Ssim. 2010 20th international conference on pattern recognition. IEEE. pp. 2366-2369.
[18] Simonyan, K., Zisserman, A., 2014. Very deep convolutional networks for large-scale image recognition. arXiv preprint arXiv:1409.1556.
Downloads
How to Cite
Issue
Article Type
License
Copyright © 2022 Author(s)

This is an open access article under the Creative Commons Attribution-NonCommercial 4.0 International (CC BY-NC 4.0) License.