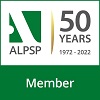
Improving U-Net Performance for Tumor Segmentation Using Attention Mechanisms
DOI:
https://doi.org/10.30564/jcsr.v6i4.7271Abstract
U-Net is a widely recognized neural network model for medical image segmentation, renowned for its efficiency in extracting features from both current and past input data. However, traditional U-Net models exhibit limitations in extracting edge features, particularly in medical CT images characterized by complex gray distributions and close pixel intervals. This leads to suboptimal performance, with low accuracy, recall, intersection over union (IoU), and F1-score. This research proposes an improved U-Net model incorporating an attention mechanism to enhance tumor segmentation accuracy and efficiency. The attention mechanism strategically weights important features, directing the network to focus on task-relevant areas. Experimental results demonstrate that our proposed attention-based U-Net model significantly improves tumor segmentation performance, achieving notable enhancements in accuracy, recall, IoU, and F1-score. Further validation across diverse datasets confirms the model's generalization ability and superiority compared to the original U-Net method. This research contributes to the advancement of medical image segmentation techniques, highlighting the potential of attention mechanisms in optimizing deep learning models for clinical applications.
Keywords:
Deep learning; Medical image segmentation; Unet model; Attention mechanismReferences
[1] Zaremba, W., Sutskever, I., Vinyals, O., 2014. Recurrent neural network regularization. arXiv preprint. arXiv:1409.2329.
[2] Ronneberger, O., Fischer, P., Brox, T., 2015. U-Net: Convolutional networks for biomedical image segmentation. In: Navab, N., Hornegger, J., Wells, W., et al. (eds) Medical Image Computing and Computer-Assisted Intervention – MICCAI 2015. MICCAI 2015. Lecture Notes in Computer Science. Springer: Cham. 9351, 234–241.
[3] Bao, W., Yue, J., Rao, Y., 2017. A deep learning framework for financial time series using stacked autoencoders and longshort term memory. PloS one. 12(7), e0180944.
[4] Bai, S., Kolter, J.Z., Koltun, V., 2018. An empirical evaluation of generic convolutional and recurrent networks for sequence modeling. arXiv preprint. arXiv:1803.01271.
[5] Jiang, H., Qiu, X., Chen, J., et al., 2019. Insulator fault detection in aerial images based on ensemble learning with multi-level perception. IEEE Access. 7, 61797–61810.
[6] Tao, X., Zhang, D., Wang, Z., et al., 2020. Detection of power line insulator defects using aerial images analyzed with convolutional neural networks. IEEE Transactions on Systems, Man, and Cybernetics: Systems. 50(4), 1486–1498.
[7] Niu, Z., Zhong, G., Yu, H., 2021. A review on the attention mechanism of deep learning. Neurocomputing. 452, 48–62.
[8] Sun, Y., Bi, F., Gao, Y., et al., 2022. A multi-attention UNet for semantic segmentation in remote sensing images. Symmetry. 14(5), 906.
[9] Peng, C., Zhang, H., Wang, Y., 2022. Fast Detection Method of Insulator String Image Basedon YOLOv3. Insulators and Surge Arresters. (01), 151–156.
Downloads
How to Cite
Issue
Article Type
License
Copyright © 2024 Author(s)

This is an open access article under the Creative Commons Attribution-NonCommercial 4.0 International (CC BY-NC 4.0) License.